Time Series Analysis and Forecasting using Python
Satyajit Pattnaik
10:05:30
Description
Learn about Time Series Analysis and Forecasting models using Python in just under 11 hours.
What You'll Learn?
- Get a solid understanding of Time Series Analysis and Forecasting
- Building different Time Series Forecasting Models in Python
- Learn about different variants of ARIMA, Facebook Prophet & LSTM models for forecasting
- 3 Industry level projects
- Understand the business scenarios where Time Series Analysis is applicable
- Use Pandas DataFrames to manipulate Time Series data and make statistical computations
Who is this for?
What You Need to Know?
More details
DescriptionIn this comprehensive Time Series Analysis and Forecasting course, you'll learn everything you need to confidently analyze time series data and make accurate predictions. Through a combination of theory and practical examples, in just 10-11 hours, you'll develop a strong foundation in time series concepts and gain hands-on experience with various models and techniques.
This course also includes Exploratory Data Analysis which might not be 100% applicable for Time Series Analysis &Â Forecasting, but these concepts are very much needed in the Data space!!
This course includes:
Understanding Time Series: Explore the fundamental concepts of time series analysis, including the different components of time series, such as trend, seasonality, and noise.
Decomposition Techniques: Learn how to decompose time series data into its individual components to better understand its underlying patterns and trends.
Autoregressive (AR) Models: Dive into autoregressive models and discover how they capture the relationship between an observation and a certain number of lagged observations.
Moving Average (MA) Models: Explore moving average models and understand how they can effectively smooth out noise and reveal hidden patterns in time series data.
ARIMA Models: Master the widely used ARIMA models, which combine the concepts of autoregressive and moving average models to handle both trend and seasonality in time series data.
Facebook Prophet: Get hands-on experience with Facebook Prophet, a powerful open-source time series forecasting tool, and learn how to leverage its capabilities to make accurate predictions.
Real-World Projects: Apply your knowledge and skills to three real-world projects, where you'll tackle various time series analysis and forecasting problems, gaining valuable experience and confidence along the way.
In addition to the objectives mentioned earlier, our course also covers the following topics:
Preprocessing and Data Cleaning: Students will learn how to preprocess and clean time series data to ensure its quality and suitability for analysis. This includes handling missing values, dealing with outliers, and performing data transformations.
Multivariate Forecasting: The course explores techniques for forecasting time series data that involve multiple variables. Students will learn how to handle and analyze datasets with multiple time series and understand the complexities and challenges associated with multivariate forecasting.
By the end of this course, you'll have a solid understanding of time series analysis and forecasting, as well as the ability to apply different models and techniques to solve real-world problems. Join us now and unlock the power of time series data to make informed predictions and drive business decisions. Enroll today and start your journey toward becoming a time series expert!
Who this course is for:
- Students need to have Python, if not, they can get started with Google Colab or any online IDEs.
- Beginner level Machine Learning concepts will be helpful
In this comprehensive Time Series Analysis and Forecasting course, you'll learn everything you need to confidently analyze time series data and make accurate predictions. Through a combination of theory and practical examples, in just 10-11 hours, you'll develop a strong foundation in time series concepts and gain hands-on experience with various models and techniques.
This course also includes Exploratory Data Analysis which might not be 100% applicable for Time Series Analysis &Â Forecasting, but these concepts are very much needed in the Data space!!
This course includes:
Understanding Time Series: Explore the fundamental concepts of time series analysis, including the different components of time series, such as trend, seasonality, and noise.
Decomposition Techniques: Learn how to decompose time series data into its individual components to better understand its underlying patterns and trends.
Autoregressive (AR) Models: Dive into autoregressive models and discover how they capture the relationship between an observation and a certain number of lagged observations.
Moving Average (MA) Models: Explore moving average models and understand how they can effectively smooth out noise and reveal hidden patterns in time series data.
ARIMA Models: Master the widely used ARIMA models, which combine the concepts of autoregressive and moving average models to handle both trend and seasonality in time series data.
Facebook Prophet: Get hands-on experience with Facebook Prophet, a powerful open-source time series forecasting tool, and learn how to leverage its capabilities to make accurate predictions.
Real-World Projects: Apply your knowledge and skills to three real-world projects, where you'll tackle various time series analysis and forecasting problems, gaining valuable experience and confidence along the way.
In addition to the objectives mentioned earlier, our course also covers the following topics:
Preprocessing and Data Cleaning: Students will learn how to preprocess and clean time series data to ensure its quality and suitability for analysis. This includes handling missing values, dealing with outliers, and performing data transformations.
Multivariate Forecasting: The course explores techniques for forecasting time series data that involve multiple variables. Students will learn how to handle and analyze datasets with multiple time series and understand the complexities and challenges associated with multivariate forecasting.
By the end of this course, you'll have a solid understanding of time series analysis and forecasting, as well as the ability to apply different models and techniques to solve real-world problems. Join us now and unlock the power of time series data to make informed predictions and drive business decisions. Enroll today and start your journey toward becoming a time series expert!
Who this course is for:
- Students need to have Python, if not, they can get started with Google Colab or any online IDEs.
- Beginner level Machine Learning concepts will be helpful
User Reviews
Rating
Satyajit Pattnaik
Instructor's Courses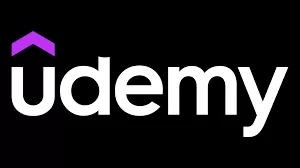
Udemy
View courses Udemy- language english
- Training sessions 79
- duration 10:05:30
- Release Date 2024/03/11