Supervised Learning - Regression Models
Elearning Moocs
13:12:46
Description
Supervised Learning - Regression Models
What You'll Learn?
- Understanding the purpose and applications of regression models in various fields, such as economics, finance.
- Exploring the basic concept of simple linear regression, where one dependent variable is modeled against a single independent variable
- Understanding how to fit polynomial functions to data, allowing for nonlinear relationships between variables.
- Extending the concepts of linear regression to multiple independent variables and learning how to interpret the coefficients of each predictor.
Who is this for?
What You Need to Know?
More details
DescriptionThe Comprehensive Regression Models course is designed to provide students with an in-depth understanding of regression analysis, one of the most widely used statistical techniques for analyzing relationships between variables. Through a combination of theoretical foundations, practical applications, and hands-on exercises, this course aims to equip students with the necessary skills to build, interpret, and validate regression models effectively. Students will gain a solid grasp of regression concepts, enabling them to make informed decisions when dealing with complex data sets and real-world scenarios. This course is intended for advanced undergraduate and graduate students, as well as professionals seeking to enhance their statistical knowledge and analytical abilities.
To ensure students can fully engage with the course material, a strong background in statistics and basic knowledge of linear algebra is recommended. Prior exposure to introductory statistics and familiarity with data analysis concepts (e.g., hypothesis testing, descriptive statistics) will be advantageous.
The Comprehensive Regression Models course empowers students to become proficient analysts and decision-makers in their academic and professional pursuits, making informed choices based on evidence and data-driven insights. Armed with this valuable skillset, graduates of this course will be better positioned to contribute meaningfully to research, policy-making, and problem-solving across various domains, enhancing their career prospects and their ability to drive positive change in the world.
Course Objectives:
Understand the Fundamentals: Students will be introduced to the fundamentals of regression analysis, including the different types of regression (e.g., linear, multiple, logistic, polynomial, etc.), assumptions, and underlying mathematical concepts. Emphasis will be placed on the interpretation of coefficients, the concept of prediction, and assessing the goodness-of-fit of regression models.
Regression Model Building: Participants will learn the step-by-step process of building regression models. This involves techniques for variable selection, handling categorical variables, dealing with collinearity, and model comparison. Students will be exposed to both automated and manual methods to ensure a comprehensive understanding of the model building process.
Model Assessment and Validation: Evaluating the performance and validity of regression models is crucial. Students will explore diagnostic tools to assess model assumptions, identify outliers, and check for heteroscedasticity.
Interpreting and Communicating Results: Being able to interpret regression results accurately and effectively communicate findings is essential. Students will learn how to interpret coefficients, measure their significance, and communicate the practical implications of the results to various stakeholders in a clear and concise manner.
Advanced Topics in Regression: The course will delve into advanced topics, including time series regression, nonlinear regression, hierarchical linear models, and generalized linear models. Students will gain insights into when and how to apply these techniques to tackle real-world challenges.
Real-world Applications: Throughout the course, students will be exposed to real-world case studies and examples from various disciplines such as economics, social sciences, healthcare, and engineering. This exposure will enable students to apply regression analysis in different contexts and understand the relevance of regression models in diverse scenarios.
Statistical Software: Hands-on experience is a critical aspect of this course. Students will work with popular statistical software packages (e.g., R, Python, or SPSS) to implement regression models and perform data analysis. By the end of the course, participants will have gained proficiency in using these tools for regression modeling.
Course Conclusion:
In conclusion, the Comprehensive Regression Models course offers an in-depth exploration of regression analysis, providing students with the necessary tools and knowledge to utilize this powerful statistical technique effectively. Throughout the course, students will gain hands-on experience with real-world datasets, ensuring they are well-equipped to apply regression analysis to a wide range of practical scenarios. By mastering regression techniques, students will be prepared to contribute to various fields, such as research, business, policy-making, and more, making data-informed decisions that lead to positive outcomes. Whether pursuing further studies or entering the workforce, graduates of this course will possess a valuable skillset that is highly sought after in today's data-driven world. As the demand for data analysis and predictive modeling continues to grow, this course will empower students to become proficient analysts and problem solvers, capable of making a significant impact in their respective domains.
By the end of this course, participants will be able to:
Understand the theoretical underpinnings of various regression models and their assumptions.
Build and validate regression models using appropriate techniques and tools.
Interpret regression results and communicate findings to different stakeholders.
Apply regression analysis to solve complex problems in diverse fields.
Confidently use statistical software for data analysis and regression modeling.
Who this course is for:
- Students and Researchers: Those studying or conducting research in fields like statistics, economics, social sciences, and data science, where regression analysis is a fundamental tool.
- Data Analysts and Data Scientists: Professionals who work with data and want to gain a deeper understanding of regression techniques to analyze relationships between variables and make predictions.
- Individuals working in business analytics, marketing, finance, or any other domain where data-driven decision-making is crucial.
- Those with programming skills who want to expand their knowledge to include regression modeling for data analysis and prediction tasks.
The Comprehensive Regression Models course is designed to provide students with an in-depth understanding of regression analysis, one of the most widely used statistical techniques for analyzing relationships between variables. Through a combination of theoretical foundations, practical applications, and hands-on exercises, this course aims to equip students with the necessary skills to build, interpret, and validate regression models effectively. Students will gain a solid grasp of regression concepts, enabling them to make informed decisions when dealing with complex data sets and real-world scenarios. This course is intended for advanced undergraduate and graduate students, as well as professionals seeking to enhance their statistical knowledge and analytical abilities.
To ensure students can fully engage with the course material, a strong background in statistics and basic knowledge of linear algebra is recommended. Prior exposure to introductory statistics and familiarity with data analysis concepts (e.g., hypothesis testing, descriptive statistics) will be advantageous.
The Comprehensive Regression Models course empowers students to become proficient analysts and decision-makers in their academic and professional pursuits, making informed choices based on evidence and data-driven insights. Armed with this valuable skillset, graduates of this course will be better positioned to contribute meaningfully to research, policy-making, and problem-solving across various domains, enhancing their career prospects and their ability to drive positive change in the world.
Course Objectives:
Understand the Fundamentals: Students will be introduced to the fundamentals of regression analysis, including the different types of regression (e.g., linear, multiple, logistic, polynomial, etc.), assumptions, and underlying mathematical concepts. Emphasis will be placed on the interpretation of coefficients, the concept of prediction, and assessing the goodness-of-fit of regression models.
Regression Model Building: Participants will learn the step-by-step process of building regression models. This involves techniques for variable selection, handling categorical variables, dealing with collinearity, and model comparison. Students will be exposed to both automated and manual methods to ensure a comprehensive understanding of the model building process.
Model Assessment and Validation: Evaluating the performance and validity of regression models is crucial. Students will explore diagnostic tools to assess model assumptions, identify outliers, and check for heteroscedasticity.
Interpreting and Communicating Results: Being able to interpret regression results accurately and effectively communicate findings is essential. Students will learn how to interpret coefficients, measure their significance, and communicate the practical implications of the results to various stakeholders in a clear and concise manner.
Advanced Topics in Regression: The course will delve into advanced topics, including time series regression, nonlinear regression, hierarchical linear models, and generalized linear models. Students will gain insights into when and how to apply these techniques to tackle real-world challenges.
Real-world Applications: Throughout the course, students will be exposed to real-world case studies and examples from various disciplines such as economics, social sciences, healthcare, and engineering. This exposure will enable students to apply regression analysis in different contexts and understand the relevance of regression models in diverse scenarios.
Statistical Software: Hands-on experience is a critical aspect of this course. Students will work with popular statistical software packages (e.g., R, Python, or SPSS) to implement regression models and perform data analysis. By the end of the course, participants will have gained proficiency in using these tools for regression modeling.
Course Conclusion:
In conclusion, the Comprehensive Regression Models course offers an in-depth exploration of regression analysis, providing students with the necessary tools and knowledge to utilize this powerful statistical technique effectively. Throughout the course, students will gain hands-on experience with real-world datasets, ensuring they are well-equipped to apply regression analysis to a wide range of practical scenarios. By mastering regression techniques, students will be prepared to contribute to various fields, such as research, business, policy-making, and more, making data-informed decisions that lead to positive outcomes. Whether pursuing further studies or entering the workforce, graduates of this course will possess a valuable skillset that is highly sought after in today's data-driven world. As the demand for data analysis and predictive modeling continues to grow, this course will empower students to become proficient analysts and problem solvers, capable of making a significant impact in their respective domains.
By the end of this course, participants will be able to:
Understand the theoretical underpinnings of various regression models and their assumptions.
Build and validate regression models using appropriate techniques and tools.
Interpret regression results and communicate findings to different stakeholders.
Apply regression analysis to solve complex problems in diverse fields.
Confidently use statistical software for data analysis and regression modeling.
Who this course is for:
- Students and Researchers: Those studying or conducting research in fields like statistics, economics, social sciences, and data science, where regression analysis is a fundamental tool.
- Data Analysts and Data Scientists: Professionals who work with data and want to gain a deeper understanding of regression techniques to analyze relationships between variables and make predictions.
- Individuals working in business analytics, marketing, finance, or any other domain where data-driven decision-making is crucial.
- Those with programming skills who want to expand their knowledge to include regression modeling for data analysis and prediction tasks.
User Reviews
Rating
Elearning Moocs
Instructor's Courses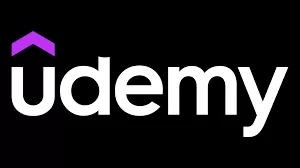
Udemy
View courses Udemy- language english
- Training sessions 67
- duration 13:12:46
- Release Date 2023/09/04