Statistics For Data Science and Machine Learning with Python
Taher Assaf
7:20:38
Description
Practical Statistics with Python for Data Science & Machine Learning Statistical Modeling Using Sci-kit Learn and Scipy
What You'll Learn?
- You will learn to use data exploratory analysis in data science.
- You will learn the most common data types such as continuous and categorical data.
- You will learn the central tendency measures and the dispersion measures in statistics.
- You will learn the concepts of population data vs sample data.
- You will learn what random sampling means and how it affects data analysis.
- You will learn about outliers and sampling errors and how they are related to data analysis.
- You will learn how to visualize data distribution using boxplots, violin plots, histograms, and density plots.
- You will learn how to visualize categorical data using bar plots and pie charts.
- You will learn how to calculate correlation and covariance between features in the dataset.
- You will learn how to visualize a correlation matrix using heat maps.
- You will learn the most common probability distributions such as normal distribution and binomial distribution.
- You will learn how to perform normality tests to check for deviation from normality.
- You will learn how to test skewed distributions in real-world data.
- You will learn how to standardize and normalize data to have the same scale.
- You will learn how to transform skewed data to be normally distributed using different transformation methods such as log, square root, and power transformation
- You will learn how to calculate confidence intervals for statistical estimates such as model accuracy.
- You will learn bootstrapping in statistics and how it is used in machine learning.
- You will learn how to evaluate machine learning models.
- You will practically understand the concepts of bias and variance in data modeling.
- You will understand what we mean by underfitting and overfitting in machine leaning and statistical modeling.
- You will learn the most common evaluation metrics for regression models in machine learning.
- You will learn the evaluation metrics for classification models.
- You will learn how to validate predictive machine learning such as regression and classification models.
- You will learn how to use different validation techniques for machine learning such as hold-out validation and cross-validation techniques.
Who is this for?
What You Need to Know?
More details
DescriptionThis course is ideal for you if you want to gain knowledge in statistical methods required for Data Science and machine learning!
Learning Statistics is an essential part of becoming a professional data scientist. Most data science learners study python for data science and ignore or postpone studying statistics. One reason for that is the lack of resources and courses that teach statistics for data science and machine learning.
Statistics is a huge field of science, but the good news for data science learners is that not all statistics are required for data science and machine learning. However, this fact makes it more difficult for learners to study statistics because they are not sure where to start and what are the most relevant topics of statistics for data science.
This course comes to close this gap.
This course is designed for both beginners with no background in statistics for data science or for those looking to extend their knowledge in the field of statistics for data science.
I have organized this course to be used as a video library for you so that you can use it in the future as a reference. Every lecture in this comprehensive course covers a single topic.
In this comprehensive course, I will guide you to learn the most common and essential methods of statistics for data analysis and data modeling.
My course is equivalent to a college-level course in statistics for data science and machine learning that usually cost thousands of dollars. Here, I give you the opportunity to learn all that information at a fraction of the cost! With 77 HD video lectures, many exercises, and two projects with solutions.
All materials presented in this course are provided in detailed downloadable notebooks for every lecture.
Most students focus on learning python codes for data science, however, this is not enough to be a proficient data scientist. You also need to understand the statistical foundation of python methods. Models and data analysis can be easily created in python, but to be able to choose the correct method or select the best model you need to understand the statistical methods that are used in these models. Here are a few of the topics that you will be learning in this comprehensive course:
· Data Types and Structures
· Exploratory Data Analysis
· Central Tendency Measures
· Dispersion Measures
· Visualizing Data Distributions
· Correlation, Scatterplots, and Heat Maps
· Data Distribution and Data Sampling
· Data Scaling and Transformation
· Data Scaling and Transformation
· Confidence Intervals
· Evaluation Metrics for Machine Learning
· Model Validation Techniques in Machine Learning
Enroll in the course and gain the essential knowledge of statistical methods for data science today!
Who this course is for:
- This course is for students who want to learn statistics from data science perspective.
This course is ideal for you if you want to gain knowledge in statistical methods required for Data Science and machine learning!
Learning Statistics is an essential part of becoming a professional data scientist. Most data science learners study python for data science and ignore or postpone studying statistics. One reason for that is the lack of resources and courses that teach statistics for data science and machine learning.
Statistics is a huge field of science, but the good news for data science learners is that not all statistics are required for data science and machine learning. However, this fact makes it more difficult for learners to study statistics because they are not sure where to start and what are the most relevant topics of statistics for data science.
This course comes to close this gap.
This course is designed for both beginners with no background in statistics for data science or for those looking to extend their knowledge in the field of statistics for data science.
I have organized this course to be used as a video library for you so that you can use it in the future as a reference. Every lecture in this comprehensive course covers a single topic.
In this comprehensive course, I will guide you to learn the most common and essential methods of statistics for data analysis and data modeling.
My course is equivalent to a college-level course in statistics for data science and machine learning that usually cost thousands of dollars. Here, I give you the opportunity to learn all that information at a fraction of the cost! With 77 HD video lectures, many exercises, and two projects with solutions.
All materials presented in this course are provided in detailed downloadable notebooks for every lecture.
Most students focus on learning python codes for data science, however, this is not enough to be a proficient data scientist. You also need to understand the statistical foundation of python methods. Models and data analysis can be easily created in python, but to be able to choose the correct method or select the best model you need to understand the statistical methods that are used in these models. Here are a few of the topics that you will be learning in this comprehensive course:
· Data Types and Structures
· Exploratory Data Analysis
· Central Tendency Measures
· Dispersion Measures
· Visualizing Data Distributions
· Correlation, Scatterplots, and Heat Maps
· Data Distribution and Data Sampling
· Data Scaling and Transformation
· Data Scaling and Transformation
· Confidence Intervals
· Evaluation Metrics for Machine Learning
· Model Validation Techniques in Machine Learning
Enroll in the course and gain the essential knowledge of statistical methods for data science today!
Who this course is for:
- This course is for students who want to learn statistics from data science perspective.
User Reviews
Rating
Taher Assaf
Instructor's Courses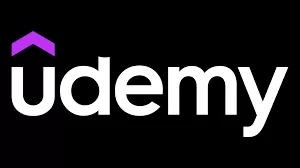
Udemy
View courses Udemy- language english
- Training sessions 74
- duration 7:20:38
- Release Date 2022/12/03