Probability and Statistics for Machine Learning
Focused View
8:57:30
76 View
00001 Probability and Statistics for Machine Learning - Introduction.mp4
03:21
00002 Topics.mp4
00:37
00003 1.1 Orientation to the Machine Learning Foundations Series.mp4
02:48
00004 1.2 What Probability Theory Is.mp4
07:04
00005 1.3 Events and Sample Spaces.mp4
04:56
00006 1.4 Multiple Observations.mp4
05:58
00007 1.5 Factorials and Combinatorics.mp4
04:49
00008 1.6 Exercises.mp4
08:52
00009 1.7 The Law of Large Numbers and the Gambler s Fallacy.mp4
11:17
00010 1.8 Probability Distributions in Statistics.mp4
05:23
00011 1.9 Bayesian versus Frequentist Statistics.mp4
08:43
00012 1.10 Applications of Probability to Machine Learning.mp4
08:47
00013 Topics.mp4
00:35
00014 2.1 Discrete and Continuous Variables.mp4
04:51
00015 2.2 Probability Mass Functions.mp4
05:56
00016 2.3 Probability Density Functions.mp4
04:09
00017 2.4 Exercises on Probability Functions.mp4
02:30
00018 2.5 Expected Value.mp4
06:21
00019 2.6 Exercises on Expected Value.mp4
04:26
00020 Topics.mp4
00:22
00021 3.1 The Mean a Measure of Central Tendency.mp4
07:19
00022 3.2 Medians.mp4
02:56
00023 3.3 Modes.mp4
06:22
00024 3.4 Quantiles - Percentiles Quartiles and Deciles.mp4
07:44
00025 3.5 Box-and-Whisker Plots.mp4
11:56
00026 3.6 Variance a Measure of Dispersion.mp4
07:45
00027 3.7 Standard Deviation.mp4
03:21
00028 3.8 Standard Error.mp4
04:53
00029 3.9 Covariance a Measure of Relatedness.mp4
12:43
00030 3.10. Correlation.mp4
07:45
00031 Topics.mp4
00:21
00032 4.1 Joint Probability Distribution.mp4
03:56
00033 4.2 Marginal Probability.mp4
05:11
00034 4.3 Conditional Probability.mp4
06:14
00035 4.4 Exercises.mp4
05:38
00036 4.5 Chain Rule of Probabilities.mp4
03:35
00037 4.6 Independent Random Variables.mp4
03:24
00038 4.7 Conditional Independence.mp4
05:01
00039 Topics.mp4
00:42
00040 5.1 Uniform.mp4
04:14
00041 5.2 Gaussian - Normal and Standard Normal.mp4
08:41
00042 5.3 The Central Limit Theorem.mp4
15:28
00043 5.4 Log-Normal.mp4
02:29
00044 5.5 Exponential and Laplace.mp4
04:45
00045 5.6 Binomial and Multinomial.mp4
08:06
00046 5.7 Poisson.mp4
03:36
00047 5.8 Mixture Distributions.mp4
03:29
00048 5.9 Preprocessing Data for Model Input.mp4
03:21
00049 5.10 Exercises.mp4
02:48
00050 Topics.mp4
00:28
00051 6.1 What Information Theory Is.mp4
01:11
00052 6.2 Self-Information Nats and Bits.mp4
06:20
00053 6.3 Shannon and Differential Entropy.mp4
07:10
00054 6.4 Kullback-Leibler Divergence and Cross-Entropy.mp4
05:29
00055 Topics.mp4
00:35
00056 7.1 Applications of Statistics to Machine Learning.mp4
09:23
00057 7.2 Review of Essential Probability Theory.mp4
12:08
00058 7.3 z-scores and Outliers.mp4
10:06
00059 7.4 Exercises on z-scores.mp4
03:58
00060 7.5 p-values.mp4
13:32
00061 7.6 Exercises on p-values.mp4
06:38
00062 Topics.mp4
00:35
00063 8.1 Single-Sample t-tests and Degrees of Freedom.mp4
10:36
00064 8.2 Independent t-tests.mp4
12:57
00065 8.3 Paired t-tests.mp4
13:30
00066 8.4 Applications to Machine Learning.mp4
04:13
00067 8.5 Exercises.mp4
07:55
00068 8.6 Confidence Intervals.mp4
10:27
00069 8.7 ANOVA - Analysis of Variance.mp4
03:09
00070 Topics.mp4
00:31
00071 9.1 The Pearson Correlation Coefficient.mp4
06:58
00072 9.2 R-squared Coefficient of Determination.mp4
02:55
00073 9.3 Correlation versus Causation.mp4
03:46
00074 9.4 Correcting for Multiple Comparisons.mp4
03:36
00075 Topics.mp4
00:45
00076 10.1 Independent versus Dependent Variables.mp4
05:23
00077 10.2 Linear Regression to Predict Continuous Values.mp4
07:16
00078 10.3 Fitting a Line to Points on a Cartesian Plane.mp4
15:45
00079 10.4 Linear Least Squares Exercise.mp4
05:09
00080 10.5 Ordinary Least Squares.mp4
13:09
00081 10.6 Categorical Dummy Features.mp4
17:45
00082 10.7 Logistic Regression to Predict Categories.mp4
13:23
00083 10.8 Open-Ended Exercises.mp4
03:58
00084 Topics.mp4
00:36
00085 11.1 Machine Learning versus Frequentist Statistics.mp4
07:03
00086 11.2 When to Use Bayesian Statistics.mp4
02:24
00087 11.3 Prior Probabilities.mp4
05:28
00088 11.4 Bayes Theorem.mp4
12:46
00089 11.5 Resources for Further Study of Probability and Statistics.mp4
02:03
00090 Probability and Statistics for Machine Learning - Summary.mp4
01:03
More details
User Reviews
Rating
average 0
Focused display
Category
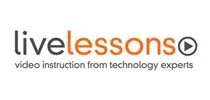
LiveLessons
View courses LiveLessonsPearson's video training library is an indispensable learning tool for today's competitive job market. Having essential technology training and certifications can open doors for career advancement and life enrichment. We take learning personally. We've published hundreds of up-to-date videos on wide variety of key topics for Professionals and IT Certification candidates. Now you can learn from renowned industry experts from anywhere in the world, without leaving home.
- language english
- Training sessions 90
- duration 8:57:30
- Release Date 2023/11/04