Optimizing Apache Spark on Databricks
Janani Ravi
2:00:05
Description
This course will teach you how to optimize the performance of Spark clusters on Azure Databricks by identifying and mitigating various issues such as data ingestion problems and performance bottlenecks
What You'll Learn?
The Apache Spark unified analytics engine is an extremely fast and performant framework for big data processing. However, you might find that your Apache Spark code running on Azure Databricks still suffers from a number of issues. These could be due to the difficulty in ingesting data in a reliable manner from a variety of sources or due to performance issues that you encounter because of disk I/O, network performance, or computation bottlenecks.
In this course, Optimizing Apache Spark on Databricks, you will first explore and understand the issues that you might encounter ingesting data into a centralized repository for data processing and insight extraction. Then, you will learn how Delta Lake on Azure Databricks allows you to store data for processing, insights, as well as machine learning on Delta tables and you will see how you can mitigate your data ingestion problems using Auto Loader on Databricks to ingest streaming data.
Next, you will explore common performance bottlenecks that you are likely to encounter while processing data in Apache Spark, issues dealing with serialization, skew, spill, and shuffle. You will learn techniques to mitigate these issues and see how you can improve the performance of your processing code using disk partitioning, z-order clustering, and bucketing.
Finally, you will learn how you can share resources on the cluster using scheduler pools and fair scheduling and how you can reduce disk read and write operations using caching on Delta tables.
When you are finished with this course, you will have the skills and knowledge of optimizing performance in Spark needed to get the best out of your Spark cluster.
More details
User Reviews
Rating
Janani Ravi
Instructor's Courses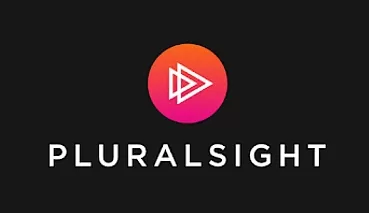
Pluralsight
View courses Pluralsight- language english
- Training sessions 30
- duration 2:00:05
- level preliminary
- Release Date 2023/12/15