Optimization (pyomo) for Energy Investments using Python
Dr. Spyros Giannelos
4:11:37
Description
Mathematical Optimization Investment models using Python (pyomo)
What You'll Learn?
- Pyomo and Python
- Mathematical Optimization models from scratch
- Energy Investment problems. Focus: Sustainable Energy. All on Python.
- The subtitles are manually created. Therefore, they are fully accurate. They are not auto-generated.
- Part of the giannelos dot com official certificate
Who is this for?
More details
Description
What is the course about:
This course teaches how to apply Mathematical Optimization in order to find the most economical (optimal) investment decisions, with an application to energy.
A Mathematical Optimization Model is a type of Data Science Model, which is used for economic analyses.
In this case, the course shows how to use such models for analyses of Investments in Energy Infrastructure.
Focus is placed on Renewables infrastructures such as Wind Farms, Solar Photovoltaics, and Hydropower units.
â
The idea of this course is that you either have your own consultancy company or you work for a consultancy company, whose clients are companies interested in investing in energy but have not yet decided when to start the construction, which location to select, and they are not sure about how much the cost will be.
âYou will build an Optimization model that will model the specific requirements of the client as accurately as possible, and produce results that you can explain to the client.
The clients will provide a number of input data (typically Excel files) that will need to be taken into account. This means that the Optimization model will have to read the input data that the client has provided, which can be done through Python.
In this course, the entire process is displayed in detail.
Who:
I am a research fellow at Imperial College London, and I have been part of high-tech projects at the intersection of Academia & Industry for over 10 years, prior to, during & after my Ph.D. I am also the founder of the giannelos dot com program in data science.
Doctor of Philosophy (Ph.D.) in Analytics & Mathematical Optimization applied to Energy Investments, from Imperial College London, and Masters of Engineering (M. Eng.) in Power Systems and Economics.
Special Acknowledgements:
To Himalaya Bir Shrestha who has been contributing to the development of Python scripts for this course and to Medium with insightful posts.
Important:
Prerequisites: The course Data Science Code that appears all the time at Workplace.
Every detail is explained, so that you won't have to search online, or guess. In the end, you will feel confident in your knowledge and skills.
We start from scratch so that you do not need to have done any preparatory work in advance at all. Just follow what is shown on screen, because we go slowly and explain everything in detail.
Who this course is for:
- Enterpreneurs
- Economists.
- Quants
- Members of the highly googled giannelos dot com program
- Investment Bankers
- Academics, PhD Students, MSc Students, Undergrads
- Postgraduate and PhD students.
- Data Scientists
- Energy professionals (investment planning, power system analysis)
- Software Engineers
- Finance professionals
What is the course about:
This course teaches how to apply Mathematical Optimization in order to find the most economical (optimal) investment decisions, with an application to energy.
A Mathematical Optimization Model is a type of Data Science Model, which is used for economic analyses.
In this case, the course shows how to use such models for analyses of Investments in Energy Infrastructure.
Focus is placed on Renewables infrastructures such as Wind Farms, Solar Photovoltaics, and Hydropower units.
â
The idea of this course is that you either have your own consultancy company or you work for a consultancy company, whose clients are companies interested in investing in energy but have not yet decided when to start the construction, which location to select, and they are not sure about how much the cost will be.
âYou will build an Optimization model that will model the specific requirements of the client as accurately as possible, and produce results that you can explain to the client.
The clients will provide a number of input data (typically Excel files) that will need to be taken into account. This means that the Optimization model will have to read the input data that the client has provided, which can be done through Python.
In this course, the entire process is displayed in detail.
Who:
I am a research fellow at Imperial College London, and I have been part of high-tech projects at the intersection of Academia & Industry for over 10 years, prior to, during & after my Ph.D. I am also the founder of the giannelos dot com program in data science.
Doctor of Philosophy (Ph.D.) in Analytics & Mathematical Optimization applied to Energy Investments, from Imperial College London, and Masters of Engineering (M. Eng.) in Power Systems and Economics.
Special Acknowledgements:
To Himalaya Bir Shrestha who has been contributing to the development of Python scripts for this course and to Medium with insightful posts.
Important:
Prerequisites: The course Data Science Code that appears all the time at Workplace.
Every detail is explained, so that you won't have to search online, or guess. In the end, you will feel confident in your knowledge and skills.
We start from scratch so that you do not need to have done any preparatory work in advance at all. Just follow what is shown on screen, because we go slowly and explain everything in detail.
Who this course is for:
- Enterpreneurs
- Economists.
- Quants
- Members of the highly googled giannelos dot com program
- Investment Bankers
- Academics, PhD Students, MSc Students, Undergrads
- Postgraduate and PhD students.
- Data Scientists
- Energy professionals (investment planning, power system analysis)
- Software Engineers
- Finance professionals
User Reviews
Rating
Dr. Spyros Giannelos
Instructor's Courses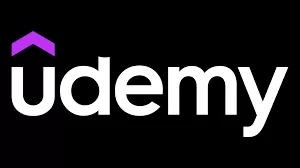
Udemy
View courses Udemy- language english
- Training sessions 52
- duration 4:11:37
- Release Date 2023/01/09