Math for Data Science Masterclass
Jose Portilla,Krista King
16:06:06
Description
Learn about probability, statistics, and more using the mathematics that are foundational to the field of data science.
What You'll Learn?
- Understand core concepts about data quality and quantity
- Learn about how to measure data with statistics
- Discover how to visualize data with a variety of plot types
- Use combinatorics to calculate permutations and combinations of objects
- Understand the key ideas in using probability to solve problems
- Learn how to use data distributions with real world data
- Discover the powerful insights from the normal distribution
- Use sampling and the central limit theorem
- Understand hypothesis testing on sample groups
- Cover the basics of linear regression
Who is this for?
More details
DescriptionWelcome to the best online course for learning about the Math behind the field of Data Science!
Working together for the first time ever, Krista King and Jose Portilla have combined forces to deliver you a best in class course experience in how to use mathematics to solve real world data science problems. This course has been specifically designed to help you understand the mathematical concepts behind the field of data science, so you can have a first principles level understanding of how to use data effectively in an organization.
Often students entering the field of data science are confused on where to start to learn about the fundamental math behind the concepts. This course was specifically designed to help bridge that gap and provide students a clear, guided path through the complex and interesting world of math used in the field of data science. Designed to balance theory and application, this is the ultimate learning experience for anyone wanting to really understand data science.
Why choose this course?
Combined together, Krista and Jose have taught over 3.2 million students about data science and mathematics and their joint expertise means you'll be able to get the best and clearest mathematical explanations from Krista with framing about real world data science applications from Jose. At the end of each section is a set of practice problems developed from real-world company situations, where you can directly apply what you know to test your understanding.
What's covered in this course?
In this course, we'll cover:
Understanding Data Concepts
Measurements of Dispersion and Central Tendency
Different ways to visualize data
Permutations
Combinatorics
Bayes' Theorem
Random Variables
Joint Distributions
Covariance and Correlation
Probability Mass and Density Functions
Binomial, Bernoulli, and Poisson Distributions
Normal Distribution and Z-Scores
Sampling and Bias
Central Limit Theorem
Hypothesis Testing
Linear Regression
and much more!
Enroll today and we'll see you inside the course!
Krista and Jose
Who this course is for:
- Anyone interested in learning more about the mathematics behind data science
Welcome to the best online course for learning about the Math behind the field of Data Science!
Working together for the first time ever, Krista King and Jose Portilla have combined forces to deliver you a best in class course experience in how to use mathematics to solve real world data science problems. This course has been specifically designed to help you understand the mathematical concepts behind the field of data science, so you can have a first principles level understanding of how to use data effectively in an organization.
Often students entering the field of data science are confused on where to start to learn about the fundamental math behind the concepts. This course was specifically designed to help bridge that gap and provide students a clear, guided path through the complex and interesting world of math used in the field of data science. Designed to balance theory and application, this is the ultimate learning experience for anyone wanting to really understand data science.
Why choose this course?
Combined together, Krista and Jose have taught over 3.2 million students about data science and mathematics and their joint expertise means you'll be able to get the best and clearest mathematical explanations from Krista with framing about real world data science applications from Jose. At the end of each section is a set of practice problems developed from real-world company situations, where you can directly apply what you know to test your understanding.
What's covered in this course?
In this course, we'll cover:
Understanding Data Concepts
Measurements of Dispersion and Central Tendency
Different ways to visualize data
Permutations
Combinatorics
Bayes' Theorem
Random Variables
Joint Distributions
Covariance and Correlation
Probability Mass and Density Functions
Binomial, Bernoulli, and Poisson Distributions
Normal Distribution and Z-Scores
Sampling and Bias
Central Limit Theorem
Hypothesis Testing
Linear Regression
and much more!
Enroll today and we'll see you inside the course!
Krista and Jose
Who this course is for:
- Anyone interested in learning more about the mathematics behind data science
User Reviews
Rating
Jose Portilla
Instructor's CoursesKrista King
Instructor's Courses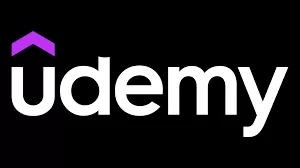
Udemy
View courses Udemy- language english
- Training sessions 59
- duration 16:06:06
- Release Date 2022/12/24