Master Machine Learning in Python with Scikit-Learn
TM Quest
8:59:53
Description
A comprehensive introduction to machine learning and data science in Python with the scikit-learn library!
What You'll Learn?
- Understand the fundamental concepts in machine learning.
- Use Python and Scikit-Learn to create machine learning models.
- Make data ready for machine learning algorithms to use.
- Critically examine machine learning models and algorithms.
- Use regression models to predict continuous values.
- Use classification models to predict classes.
- Use unsupervised algorithms to cluster data.
Who is this for?
What You Need to Know?
More details
DescriptionDo you want to get started with machine learning and data science in Python? This course is both a comprehensive and hands-on introduction to machine learning! We will use Scikit-Learn, which is the most awesome library in Python for machine learning!
What this course is all about:
In this course, we will teach you the ins and outs of both machine learning in general, as well as the Python library Scikit-Learn. Scikit-Learn is not only super popular but is also incredibly powerful for many machine learning tasks. If you are interested in machine learning in general, or specifically in Scikit-Learn, then this is the course for you. The course will teach you everything you need to know to professionally use Scikit-Learn for machine learning. We will start with the basics, and then gradually move on to more complicated topics.
Why choose us?
This course is a comprehensive introduction to machine learning in Python by using Scikit-Learn! We don't shy away from the technical stuff and want you to stand out with your newly learned Scikit-Learn skills.
The course is filled with carefully made exercises that will reinforce the topics we teach. In between videos, we give small exercises that help you reinforce the material. Additionally, we have larger exercises where you will be given a Jupiter Notebook sheet and asked to solve a series of questions that revolve around a single topic. The exercises include data processing and cleaning, making them much closer to real-life machine learning.
We're a couple (Eirik and Stine) who love to create high-quality courses! Eirik has used Scikit-Learn professional as a data scientist, while Stine has experience with teaching programming at the university level. We both love Scikit-Learn and can't wait to teach you all about it!
Topics we will cover:
We will cover a lot of different topics in this course. In order of appearance, they are:
Introduction to Scikit-Learn
Linear Regression
Logistic Regression
Preprocessing and Pipelines
Polynomial Regression
Decision Trees and Random Forests
Cross-Validation
Regularization Techniques
Support Vector Machines
Dimensionality Reduction & PCA
Basics of Neural Networks
Supervised and Unsupervised Learning
and much more! By completing our course, you will be comfortable with both machine learning and the Python library Scikit-Learn. This gives you a great starting point for working professionally with machine learning.
Still not decided?
The course has a 30-day refund policy, so if you are unhappy with the course, then you can get your money back painlessly. If are still uncertain after reading this, then take a look at some of the free previews and see if you enjoy them. Hope to see you soon!
Who this course is for:
- Anyone who wants to break into machine learning and data science
- Beginner Python developers curious about machine learning
Do you want to get started with machine learning and data science in Python? This course is both a comprehensive and hands-on introduction to machine learning! We will use Scikit-Learn, which is the most awesome library in Python for machine learning!
What this course is all about:
In this course, we will teach you the ins and outs of both machine learning in general, as well as the Python library Scikit-Learn. Scikit-Learn is not only super popular but is also incredibly powerful for many machine learning tasks. If you are interested in machine learning in general, or specifically in Scikit-Learn, then this is the course for you. The course will teach you everything you need to know to professionally use Scikit-Learn for machine learning. We will start with the basics, and then gradually move on to more complicated topics.
Why choose us?
This course is a comprehensive introduction to machine learning in Python by using Scikit-Learn! We don't shy away from the technical stuff and want you to stand out with your newly learned Scikit-Learn skills.
The course is filled with carefully made exercises that will reinforce the topics we teach. In between videos, we give small exercises that help you reinforce the material. Additionally, we have larger exercises where you will be given a Jupiter Notebook sheet and asked to solve a series of questions that revolve around a single topic. The exercises include data processing and cleaning, making them much closer to real-life machine learning.
We're a couple (Eirik and Stine) who love to create high-quality courses! Eirik has used Scikit-Learn professional as a data scientist, while Stine has experience with teaching programming at the university level. We both love Scikit-Learn and can't wait to teach you all about it!
Topics we will cover:
We will cover a lot of different topics in this course. In order of appearance, they are:
Introduction to Scikit-Learn
Linear Regression
Logistic Regression
Preprocessing and Pipelines
Polynomial Regression
Decision Trees and Random Forests
Cross-Validation
Regularization Techniques
Support Vector Machines
Dimensionality Reduction & PCA
Basics of Neural Networks
Supervised and Unsupervised Learning
and much more! By completing our course, you will be comfortable with both machine learning and the Python library Scikit-Learn. This gives you a great starting point for working professionally with machine learning.
Still not decided?
The course has a 30-day refund policy, so if you are unhappy with the course, then you can get your money back painlessly. If are still uncertain after reading this, then take a look at some of the free previews and see if you enjoy them. Hope to see you soon!
Who this course is for:
- Anyone who wants to break into machine learning and data science
- Beginner Python developers curious about machine learning
User Reviews
Rating
TM Quest
Instructor's Courses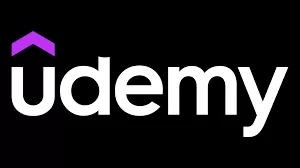
Udemy
View courses Udemy- language english
- Training sessions 94
- duration 8:59:53
- Release Date 2023/09/10