Machine Learning: Generative Adversarial Networks (GANS)
Maxime Vandegar
1:29:08
Description
Generative Adversarial Networks (GANs) | Applications | How they work | PyTorch implementation | Image generation
What You'll Learn?
- Opportunities offered by generative models
- How GANs work (intuitive & mathematical)
- Implementing a GAN from scratch
- Synthetic image generation with GANs
- Dive in the paper "Generative Adversarial Networks"
- Implementation and optimization of neural networks with PyTorch
Who is this for?
What You Need to Know?
More details
DescriptionIn this crash course, we will discuss the opportunities that generative models offer, and more specifically Generative Adversarial Networks (GANs).
I will explain how GANs work intuitively, and then we will dive into the paper that introduced them in 2014 (Ian J. Goodfellow et al.). You will therefore understand how they work in a mathematical way, which will give you the foundation to implement your first GAN from scratch.
We will implement in approximately 100 lines of code a generator, a discriminator and the pseudo-code described in the paper in order to train them. We will use the Python programming language and the PyTorch framework. After training, the generator will allow us to generate synthetic images that are indistinguishable from real images.
I believe that a concept is learned by doing and this crash course aims to give you the necessary basis to continue your learning of Machine Learning, PyTorch and generative models (GANS, Variational Autoencoders, Normalizing Flows, Diffusion Models, ...).
At the end of this course, the participant will be able to use Python (and more particularly the PyTorch framework) to implement scientific papers and artificial intelligence solutions. This course is also intended to be a stepping stone in your learning of generative models.
Beyond GANs, this course is also a general introduction to the PyTorch framework and an intermediate level Machine learning course .
Concepts covered:
The PyTorch framework in order to implement and optimize neural networks.
The use of generative models in the research and industrial world.
GANs in an intuitive way. GANs in a mathematical way.
The generation of synthetic data (such as images).
The implementation of a scientific paper.
Don't wait any longer before jumping into the world of generative models!
Who this course is for:
- Professionals who want to use the opportunities offered by generative models
- Anyone interested in generative models and GANs
- Anyone interested in machine learning & artificial intelligence
- Anyone who would like to learn PyTorch through practise
In this crash course, we will discuss the opportunities that generative models offer, and more specifically Generative Adversarial Networks (GANs).
I will explain how GANs work intuitively, and then we will dive into the paper that introduced them in 2014 (Ian J. Goodfellow et al.). You will therefore understand how they work in a mathematical way, which will give you the foundation to implement your first GAN from scratch.
We will implement in approximately 100 lines of code a generator, a discriminator and the pseudo-code described in the paper in order to train them. We will use the Python programming language and the PyTorch framework. After training, the generator will allow us to generate synthetic images that are indistinguishable from real images.
I believe that a concept is learned by doing and this crash course aims to give you the necessary basis to continue your learning of Machine Learning, PyTorch and generative models (GANS, Variational Autoencoders, Normalizing Flows, Diffusion Models, ...).
At the end of this course, the participant will be able to use Python (and more particularly the PyTorch framework) to implement scientific papers and artificial intelligence solutions. This course is also intended to be a stepping stone in your learning of generative models.
Beyond GANs, this course is also a general introduction to the PyTorch framework and an intermediate level Machine learning course .
Concepts covered:
The PyTorch framework in order to implement and optimize neural networks.
The use of generative models in the research and industrial world.
GANs in an intuitive way. GANs in a mathematical way.
The generation of synthetic data (such as images).
The implementation of a scientific paper.
Don't wait any longer before jumping into the world of generative models!
Who this course is for:
- Professionals who want to use the opportunities offered by generative models
- Anyone interested in generative models and GANs
- Anyone interested in machine learning & artificial intelligence
- Anyone who would like to learn PyTorch through practise
User Reviews
Rating
Maxime Vandegar
Instructor's Courses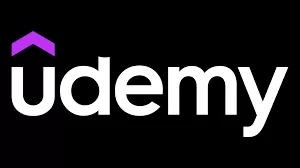
Udemy
View courses Udemy- language english
- Training sessions 12
- duration 1:29:08
- Release Date 2024/05/05