Learn Web Application Development with Machine Learning
Sachin Kafle
18:01:50
Description
Learn basic to advanced Machine Learning algorithms by creating web applications using Flask!!
What You'll Learn?
- Master Machine Learning on Python
- Learn about Regression, Classification tasks
- Learn about neural networks
- Learn about Deep neural networks with projects
- Create web applications using flask
- Simple Model building with Scikit-Learn , TensorFlow and Keras
- Creating REST API for Machine Learning models
- Learn about Exploratory Data Analysis
- Implement linear, logistic regression
- Implement convolution neural network
- Learn about Postman to test API endpoints
Who is this for?
What You Need to Know?
More details
DescriptionMachine learning is a branch of artificial intelligence (AI) focused on building applications that learn from data and improve their accuracy over time without being programmed to do so.
In data science, an algorithm is a sequence of statistical processing steps. In machine learning, algorithms are 'trained' to find patterns and features in massive amounts of data in order to make decisions and predictions based on new data. The better the algorithm, the more accurate the decisions and predictions will become as it processes more data.
Machine learning has led to some amazing results, like being able to analyze medical images and predict diseases on-par with human experts.
Google's AlphaGo program was able to beat a world champion in the strategy game go using deep reinforcement learning.
Machine learning is even being used to program self driving cars, which is going to change the automotive industry forever. Imagine a world with drastically reduced car accidents, simply by removing the element of human error.
Topics covered in this course:
1. Warm-up with Machine learning Libraries:Â numpy, pandas
2. Implement Machine Learning algorithms:Â Linear, Logistic Regression
3. Implement Neural Network from scratch
4. Introduction to Tensorflow and Keras
5. Start with simple "Hello World" flask application
6. Create flask application to implement linear regression and test the API's endpoints
7. Implement transfer learning and built an app to implement image classification
Who this course is for:
- Programmer who wants to learn machine learning by creating web applications
- Data Scientists who want to know how to test & monitor their models beyond
- Beginner Python programmer
- Machine Learning engineer who wants to create fun projects using their basic skills
Machine learning is a branch of artificial intelligence (AI) focused on building applications that learn from data and improve their accuracy over time without being programmed to do so.
In data science, an algorithm is a sequence of statistical processing steps. In machine learning, algorithms are 'trained' to find patterns and features in massive amounts of data in order to make decisions and predictions based on new data. The better the algorithm, the more accurate the decisions and predictions will become as it processes more data.
Machine learning has led to some amazing results, like being able to analyze medical images and predict diseases on-par with human experts.
Google's AlphaGo program was able to beat a world champion in the strategy game go using deep reinforcement learning.
Machine learning is even being used to program self driving cars, which is going to change the automotive industry forever. Imagine a world with drastically reduced car accidents, simply by removing the element of human error.
Topics covered in this course:
1. Warm-up with Machine learning Libraries:Â numpy, pandas
2. Implement Machine Learning algorithms:Â Linear, Logistic Regression
3. Implement Neural Network from scratch
4. Introduction to Tensorflow and Keras
5. Start with simple "Hello World" flask application
6. Create flask application to implement linear regression and test the API's endpoints
7. Implement transfer learning and built an app to implement image classification
Who this course is for:
- Programmer who wants to learn machine learning by creating web applications
- Data Scientists who want to know how to test & monitor their models beyond
- Beginner Python programmer
- Machine Learning engineer who wants to create fun projects using their basic skills
User Reviews
Rating
Sachin Kafle
Instructor's Courses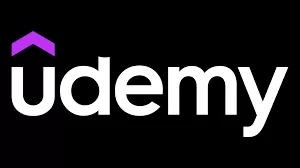
Udemy
View courses Udemy- language english
- Training sessions 91
- duration 18:01:50
- English subtitles has
- Release Date 2022/11/20