Learn Deep Reinforcement Learning Fast
Dibya Chakravorty
2:25:22
Description
From basic concepts to implementation using Ray RLlib in just 4 hours
What You'll Learn?
- Core concepts of Reinforcement Learning like environment, action, cumulative reward maximization, etc.
- Case studies of Reinforcement Learning applications in the industry
- When to apply Reinforcement Learning and when not to
- The OpenAI Gym environment API
- How to control agents inside OpenAI Gym environments
- How to use Ray RLlib to solve various learning tasks using popular algorithms PPO, DQN, TD3, SAC, etc.
- How to visualize the agent's learning behavior in Tensorboard (useful for troubleshooting)
- How to save and use the trained agent
- How to pick the right Deep RL algorithm for a given problem
Who is this for?
What You Need to Know?
More details
DescriptionUsed Keras or PyTorch? These frameworks make it easy to build Deep Neural Networks.
New Deep Reinforcement Learning frameworks like Ray RLlib make it similarly easy to build Deep RL agents. Using Ray RLlib, it's possible to prototype Deep RL agents in hours instead of days.
This course will show you how to do that. We will start from scratch, and after a few evenings of lessons and exercises, you will be able to code powerful Deep RL agents using Ray RLlib to solve various OpenAI Gym environments. This is the fastest way to get a feeling for Deep Reinforcement Learning.
We will cover the following topics in the course.
Core concepts of Reinforcement Learning like environment, action, cumulative reward maximization, etc.
Case studies of Reinforcement Learning applications in the industry
How to decide whether to use Reinforcement Learning or conventional methods for a given learning task
How to control agents inside OpenAI Gym environment (a Gym environment is just a simulation of a learning task)
How to use the industry-leading Deep Reinforcement Learning framework Ray RLlib to solve OpenAI Gym environments
Visualizing the agent's learning behavior in Tensorboard (useful for troubleshooting)
Saving and using the trained agent
How to choose the best Deep RL algorithm for a given problem
The course follows the learning-by-doing approach. This means that I will write code to solve an example problem and explain the concepts along the way in the right context. In the guided coding exercises, you will be challenged to apply what you have learned. This will ensure that you are learning applicable skills.
Here are some other features of this course.
The course consists of short videos with no fluff (on average, 6 minutes long). The entire course can be completed in 4 to 8 hours (including exercises).
The videos have high-quality English captions.
The lessons are often followed by quizzes and coding exercises so that you can test your knowledge.
The exercises are part of an overarching project, where we teach a robot how to walk. We will record a video of this agent at the end of the course, making it easy to share your new skills with others (if you wish).
This course was reviewed by a few experts and this is what they said:
"This course broke down complex RL concepts into small pieces that I could easily understand" - Martin Musiol, Managing Data Scientist at IBM
"Brilliant introduction to RL concepts and how they map to RLlib." - Jules Damji, Developer Advocate at Anyscale (creators of Ray RLlib)
Who this course is for:
- Data Scientists and Machine learning engineers who want to learn the basics of Deep Reinforcement Learning and get familiar with a production-grade Deep RL framework within a short time frame
- Technical managers who want to know how Deep RL is applied in the industry and have an overview of the standard Deep RL toolchain
- Students in a university-level Machine Learning curriculum, who want a hands-on, practical introduction to Deep Reinforcement Learning
Used Keras or PyTorch? These frameworks make it easy to build Deep Neural Networks.
New Deep Reinforcement Learning frameworks like Ray RLlib make it similarly easy to build Deep RL agents. Using Ray RLlib, it's possible to prototype Deep RL agents in hours instead of days.
This course will show you how to do that. We will start from scratch, and after a few evenings of lessons and exercises, you will be able to code powerful Deep RL agents using Ray RLlib to solve various OpenAI Gym environments. This is the fastest way to get a feeling for Deep Reinforcement Learning.
We will cover the following topics in the course.
Core concepts of Reinforcement Learning like environment, action, cumulative reward maximization, etc.
Case studies of Reinforcement Learning applications in the industry
How to decide whether to use Reinforcement Learning or conventional methods for a given learning task
How to control agents inside OpenAI Gym environment (a Gym environment is just a simulation of a learning task)
How to use the industry-leading Deep Reinforcement Learning framework Ray RLlib to solve OpenAI Gym environments
Visualizing the agent's learning behavior in Tensorboard (useful for troubleshooting)
Saving and using the trained agent
How to choose the best Deep RL algorithm for a given problem
The course follows the learning-by-doing approach. This means that I will write code to solve an example problem and explain the concepts along the way in the right context. In the guided coding exercises, you will be challenged to apply what you have learned. This will ensure that you are learning applicable skills.
Here are some other features of this course.
The course consists of short videos with no fluff (on average, 6 minutes long). The entire course can be completed in 4 to 8 hours (including exercises).
The videos have high-quality English captions.
The lessons are often followed by quizzes and coding exercises so that you can test your knowledge.
The exercises are part of an overarching project, where we teach a robot how to walk. We will record a video of this agent at the end of the course, making it easy to share your new skills with others (if you wish).
This course was reviewed by a few experts and this is what they said:
"This course broke down complex RL concepts into small pieces that I could easily understand" - Martin Musiol, Managing Data Scientist at IBM
"Brilliant introduction to RL concepts and how they map to RLlib." - Jules Damji, Developer Advocate at Anyscale (creators of Ray RLlib)
Who this course is for:
- Data Scientists and Machine learning engineers who want to learn the basics of Deep Reinforcement Learning and get familiar with a production-grade Deep RL framework within a short time frame
- Technical managers who want to know how Deep RL is applied in the industry and have an overview of the standard Deep RL toolchain
- Students in a university-level Machine Learning curriculum, who want a hands-on, practical introduction to Deep Reinforcement Learning
User Reviews
Rating
Dibya Chakravorty
Instructor's Courses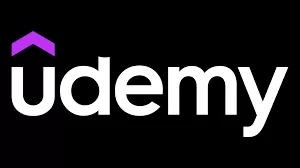
Udemy
View courses Udemy- language english
- Training sessions 21
- duration 2:25:22
- English subtitles has
- Release Date 2024/04/14