Implementing Machine Learning Workflow with RapidMiner
Janani Ravi
2:23:45
Description
In this course, you will learn how you can develop your machine learning workflow using RapidMiner Studio, a data science platform for data preparation, machine learning, and predictive model deployment.
What You'll Learn?
RapidMiner Studio provides an integrated development environment for data visualization, data preparation, machine learning, and deployment. In this course, Implementing Machine Learning Workflow with RapidMiner, you will get an overview of how you can use drag-n-drop operators to build and train machine learning models.
First, you will get introduced to RapidMiner studio, which is a no-code technology to develop your machine learning workflow. You will perform exploratory data analysis using RapidMiner, build linear regression models, evaluate models using cross-validation, and perform feature selection and normalization of input data, without writing a single line of code.
Next, you will explore a native Java library for traditional machine learning models. The Java Statistical Analysis Tool, or JSAT library, is a pure Java library that allows you to train regression, classification, and clustering models. You will use JSAT to perform linear regression, perform classification using logistic regression and decision trees, perform clustering using k-means clustering, and deploy your model using the SpringBoot framework in a limited production environment.
Finally, you will see how you can use the Deep Java Library, or DJL, to train neural network models in Java. DJL provides a native Java API and can run your training on multiple backends such as Apache MXNet, TensorFlow, and PyTorch. You will also leverage transfer learning and use pre-trained models for image classification, image segmentation, and natural language processing.
When you are finished with this course, you will be able to use no-code technologies and native Java libraries to build and train machine learning models.
More details
User Reviews
Rating
Janani Ravi
Instructor's Courses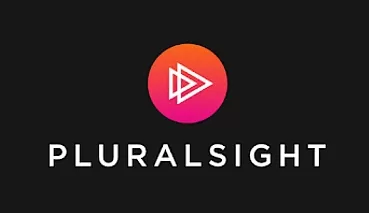
Pluralsight
View courses Pluralsight- language english
- Training sessions 36
- duration 2:23:45
- level average
- English subtitles has
- Release Date 2023/07/10