Fraud Detection using Python
Richard Ball, PhD
2:05:52
Description
Build an effective machine learning project to detect instances of financial crime.
What You'll Learn?
- Use Python to analyze a sample credit card fraud data set
- Train and improve various supervised machine learning models to detect fraud
- Generate and interpret performance metrics relevant to fraud detection
- Select an optimal classification model based on various criteria
- Apply various strategies for improving the performance of your fraud detection models
Who is this for?
More details
DescriptionIf you're interested in detecting fraud using machine learning, then this course is for you!
Fraud is a massive problem for many modern organizations, as bad actors are becoming increasingly sophisticated both in methodology and technical ability. Detecting fraud is therefore an important problem that is never going to be completely solved. By taking this course, you'll be levelling up with a hireable skillset that is likely going to be relevant and for many years to come.
This course was developed by myself, a Principal Data Scientist with a PhD in Machine Learning and real-world expertise in deploying production machine learning models for detecting fraud in the financial services industry.
In this course, students will be introduced to the problem of fraud in industry, and how it can be solved via the introduction of various machine learning approaches. I will walk you through an example fraud detection problem, where you will get hands-on exposure to building models using Python. This will include navigating the challenging problem of fraud, where special consideration needs to be given to the highly imbalanced nature of the data.
The lessons covered in this course include:
Lesson 1 - Introduction to fraud detection: anomaly detection, class imbalance
Lesson 2 - Training a supervised machine learning model to detect fraud: logistic regression, XGBoost, performance improvement through hyperparameter optimization
Lesson 3 - Performance metrics for fraud detection: confusion matrix, cost of misclassification, accuracy paradox, implementing metrics in scikit-learn
Lesson 4 - Optimal model selection: threshold optimization using performance metrics, threshold optimization using cost of fraud, introduction to Streamlit, building a threshold simulator for visual inspection
Lesson 5 - Strategies for improving model performance: sampling techniques
Each lesson builds on the practical knowledge achieved in the prior lessons, allowing for students to produce a completed end-to-end project as the final output of the course. This project could serve as an important part of a student's portfolio of projects, assisting with their job search and professional development endeavors.
The Python technology stack used within this course includes the following: pandas, numpy, matplotlib, scikit-learn, seaborn, XGBoost, Streamlit and imblearn.
Who this course is for:
- Any fraud analysts interested in machine learning.
- Anyone interested in learning about how to apply machine learning to detect fraud.
- Data scientists looking to train a fraud detection model for their organizations.
If you're interested in detecting fraud using machine learning, then this course is for you!
Fraud is a massive problem for many modern organizations, as bad actors are becoming increasingly sophisticated both in methodology and technical ability. Detecting fraud is therefore an important problem that is never going to be completely solved. By taking this course, you'll be levelling up with a hireable skillset that is likely going to be relevant and for many years to come.
This course was developed by myself, a Principal Data Scientist with a PhD in Machine Learning and real-world expertise in deploying production machine learning models for detecting fraud in the financial services industry.
In this course, students will be introduced to the problem of fraud in industry, and how it can be solved via the introduction of various machine learning approaches. I will walk you through an example fraud detection problem, where you will get hands-on exposure to building models using Python. This will include navigating the challenging problem of fraud, where special consideration needs to be given to the highly imbalanced nature of the data.
The lessons covered in this course include:
Lesson 1 - Introduction to fraud detection: anomaly detection, class imbalance
Lesson 2 - Training a supervised machine learning model to detect fraud: logistic regression, XGBoost, performance improvement through hyperparameter optimization
Lesson 3 - Performance metrics for fraud detection: confusion matrix, cost of misclassification, accuracy paradox, implementing metrics in scikit-learn
Lesson 4 - Optimal model selection: threshold optimization using performance metrics, threshold optimization using cost of fraud, introduction to Streamlit, building a threshold simulator for visual inspection
Lesson 5 - Strategies for improving model performance: sampling techniques
Each lesson builds on the practical knowledge achieved in the prior lessons, allowing for students to produce a completed end-to-end project as the final output of the course. This project could serve as an important part of a student's portfolio of projects, assisting with their job search and professional development endeavors.
The Python technology stack used within this course includes the following: pandas, numpy, matplotlib, scikit-learn, seaborn, XGBoost, Streamlit and imblearn.
Who this course is for:
- Any fraud analysts interested in machine learning.
- Anyone interested in learning about how to apply machine learning to detect fraud.
- Data scientists looking to train a fraud detection model for their organizations.
User Reviews
Rating
Richard Ball, PhD
Instructor's Courses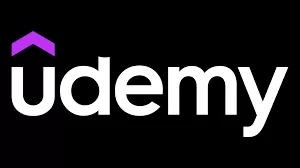
Udemy
View courses Udemy- language english
- Training sessions 20
- duration 2:05:52
- Release Date 2023/02/28