Deep Learning for Beginners: Core Concepts and PyTorch
Seungchan Lee,Nami Kim
9:39:44
Description
Get an Intuitive Understanding of Deep Learning and Artificial Intelligence
What You'll Learn?
- Develop an intuitive understanding of Deep Learning
- Visual and intuitive understanding of core math concepts behind Deep Learning
- Detailed view of how exactly deep neural networks work beneath the hood
- Computational graphs (which libraries like PyTorch and Tensorflow are built on)
- Build neural networks from scratch using PyTorch and PyTorch Lightening
- You’ll be ready to explore the cutting edge of AI and more advanced neural networks like CNNs, RNNs and Transformers
- You'll be able to understand what deep learning experts are talking about in articles and interviews
- You’ll be able to start experimenting with your own AI projects using PyTorch
Who is this for?
What You Need to Know?
More details
DescriptionAre you interested in Artificial Intelligence (AI), Machine Learning and Artificial Neural Network?
Are you afraid of getting started with Deep Learning because it sounds too technical?
Have you been watching Deep Learning videos, but still donât feel like you âgetâ it?
Iâve been there myself! I donât have an engineering background. I learned to code on my own. But AI still seemed completely out of reach.
This course was built to save you many months of frustration trying to decipher Deep Learning. After taking this course, youâll feel ready to tackle more advanced, cutting-edge topics in AI.
In this course:
We assume as little prior knowledge as possible. No engineering or computer science background required (except for basic Python knowledge). You donât know all the math needed for Deep Learning? Thatâs OK. We'll go through them all together - step by step.
We'll "reinvent" a deep neural network so you'll have an intimate knowledge of the underlying mechanics. This will make you feel more comfortable with Deep Learning and give you an intuitive feel for the subject.
We'll also build a basic neural network from scratch in PyTorch and PyTorch Lightning and train an MNIST model for handwritten digit recognition.
After taking this course:
Youâll finally feel you have an âintuitiveâ understanding of Deep Learning and feel confident expanding your knowledge further.
If you go back to the popular courses you had trouble understanding before (like Andrew Ng's courses or Jeremy Howards' Fastai course), youâll be pleasantly surprised at how much more you can understand.
You'll be able to understand what experts like Geoffrey Hinton are saying in articles or Andrej Karpathy is saying during Tesla Autonomy Day.
You'll be well equipped with both practical and theoretical understanding to start exploring more advanced neural network architectures like Convolutional Neural Network (CNN), Recurrent Neural Network (RNN), transformers, etc. and start your journey towards the cutting edge of AI, Supervised and Unsupervised learning, and more.
You can start experimenting with your own AI projects using PyTorch and Supervised Learning
This course is perfect for you if you are:
Interested in Deep Learning and PyTorch but struggling with the core concepts
Someone from a non-engineering background transitioning into an engineering career
Familiar with the basics but wish explore more advanced knowledge.
Already working with Deep Learning models, but want to supercharge your understanding
A Python Developer, looking to advance your career
This 9.5 hour course will teach you all the basic concepts as well as the application of your knowledge. You get 40 downloadable resources, full lifetime access, 30-Day Money-Back Guarantee and a Certificate of Completion.
So what stops you from taking a deep dive into the amazing world of Deep Learning?
Who this course is for:
- Students who want learn Deep Learning for the first time
- Beginners who want to finally understand Deep Learning at an intuitive level
- Professionals looking to supercharge their understanding of Deep Learning fundamentals
Are you interested in Artificial Intelligence (AI), Machine Learning and Artificial Neural Network?
Are you afraid of getting started with Deep Learning because it sounds too technical?
Have you been watching Deep Learning videos, but still donât feel like you âgetâ it?
Iâve been there myself! I donât have an engineering background. I learned to code on my own. But AI still seemed completely out of reach.
This course was built to save you many months of frustration trying to decipher Deep Learning. After taking this course, youâll feel ready to tackle more advanced, cutting-edge topics in AI.
In this course:
We assume as little prior knowledge as possible. No engineering or computer science background required (except for basic Python knowledge). You donât know all the math needed for Deep Learning? Thatâs OK. We'll go through them all together - step by step.
We'll "reinvent" a deep neural network so you'll have an intimate knowledge of the underlying mechanics. This will make you feel more comfortable with Deep Learning and give you an intuitive feel for the subject.
We'll also build a basic neural network from scratch in PyTorch and PyTorch Lightning and train an MNIST model for handwritten digit recognition.
After taking this course:
Youâll finally feel you have an âintuitiveâ understanding of Deep Learning and feel confident expanding your knowledge further.
If you go back to the popular courses you had trouble understanding before (like Andrew Ng's courses or Jeremy Howards' Fastai course), youâll be pleasantly surprised at how much more you can understand.
You'll be able to understand what experts like Geoffrey Hinton are saying in articles or Andrej Karpathy is saying during Tesla Autonomy Day.
You'll be well equipped with both practical and theoretical understanding to start exploring more advanced neural network architectures like Convolutional Neural Network (CNN), Recurrent Neural Network (RNN), transformers, etc. and start your journey towards the cutting edge of AI, Supervised and Unsupervised learning, and more.
You can start experimenting with your own AI projects using PyTorch and Supervised Learning
This course is perfect for you if you are:
Interested in Deep Learning and PyTorch but struggling with the core concepts
Someone from a non-engineering background transitioning into an engineering career
Familiar with the basics but wish explore more advanced knowledge.
Already working with Deep Learning models, but want to supercharge your understanding
A Python Developer, looking to advance your career
This 9.5 hour course will teach you all the basic concepts as well as the application of your knowledge. You get 40 downloadable resources, full lifetime access, 30-Day Money-Back Guarantee and a Certificate of Completion.
So what stops you from taking a deep dive into the amazing world of Deep Learning?
Who this course is for:
- Students who want learn Deep Learning for the first time
- Beginners who want to finally understand Deep Learning at an intuitive level
- Professionals looking to supercharge their understanding of Deep Learning fundamentals
User Reviews
Rating
Seungchan Lee
Instructor's CoursesNami Kim
Instructor's Courses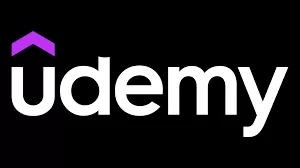
Udemy
View courses Udemy- language english
- Training sessions 55
- duration 9:39:44
- English subtitles has
- Release Date 2024/03/11