Data Science Methodology in Action using ikigailabs
Neena Sathi
4:46:57
Description
Gain hands-on experience in building a Data Driven AI engagement using ikigailabs
What You'll Learn?
- Students will learn proven data science methodology to deal with big data challenges as we move from BI world to AI world.
- Students will use real case study and will gain hands-on experience in Designing / prototyping a Data science engagement on the chosen case study.
- We divide the data scientists into clickers and coders. Clickers Examples include SPSS Modeler, Excel, and ikigailabs. This course is primarily for clickers
- This course uses ikigailabs as a tool to show all necessary steps and activities needed for data science engagement.
Who is this for?
What You Need to Know?
More details
DescriptionWith explosive growth of data in unstructured data, we have ample opportunities to design, develop and deploy AI models. While there are many courses which teach you Data Science, you need a step-by-step guide on how to select a problem, explore data, develop & deploy models, and improve the model using user feedback and learning. This course covers many big data challenges and modifies CRISP-DM to deal with big data. This course provides you a methodology for AI model development and deployment as modified by us to deal with AI and big data. Our modifications have been tried on many real-life large-scale projects. We will select a real case study for this data science project and will provide hands-on experience in Designing / prototyping a Data science engagement on the chosen case study. You will be able to use the results in your day-to-day life.
We divide the data scientists into clickers and coders. Clickers are those data scientists who use a data science tool with a user interface to provide a high-level specification. Examples include SPSS Modeler, Excel, Alteryx and Ikigailabs. In each case you can add formula using pre-packaged libraries without writing code. The second set of data scientists are those who use a procedural language with libraries to write code for data science work. The objective of this course is to get you an introductory clicker experience in data science using Ikigailabs as a tool.
Related courses:
· If you are interested in a coding course, we offer a course using Python.
· Our data science methodology course is also designed for Business Analysts and Project Managers with limited development background.
· If you are interested in advanced machine learning, we have made recommendation for courses.
· If you are interested in model deployment, monitoring and control, we offer a course on AI governance and control.
Course starts with two critical activities
· Set up Environment - step by step instructions in preparing sandbox environment for your Ikigailabs exercises
· Data Science Methodology - to review key steps, tasks and activities associated with our data science methodology
After above section, this course introduces our 7-step data science methodology and use Python to explain each step using our real-life use case example. These 7 steps include
· Step 1: Describe Use Case to explain selected use case for data science work
· Step 2: Describe Data to describe Data Sources and explain data sets using Ikigailabs
· Step 3: Prepare Datasets to Prepare Data Sets using Ikigailabs
· Step 4: Develop Model will provide hands-on exercises in applying many AI modeling techniques on data sets such as clustering, and regression using Ikigailabs.
· Step 5: Evaluate Model will provide measurements to Evaluate your AI Model Results
· Step 6: Deploy Model will provide process for deploying your AI models.
· Step 7: Monitor model will provide process for continuous monitoring and evaluating your models in production
In this course, we will give you an opportunity to design a use case and then work on its implementation using Ikigailabs. You should download all data sets and sample code. Complete all assignment in each section of the course and submit your final notebook using instructions provided.
Who this course is for:
- This course is for anyone interested in becoming a data scientist such as students, business analysts, developers, testing professionals.
- There are several job categories where this course can be used as introductory material, such as data scientists, AI or automation engineer, test engineers, and knowledge engineers.
With explosive growth of data in unstructured data, we have ample opportunities to design, develop and deploy AI models. While there are many courses which teach you Data Science, you need a step-by-step guide on how to select a problem, explore data, develop & deploy models, and improve the model using user feedback and learning. This course covers many big data challenges and modifies CRISP-DM to deal with big data. This course provides you a methodology for AI model development and deployment as modified by us to deal with AI and big data. Our modifications have been tried on many real-life large-scale projects. We will select a real case study for this data science project and will provide hands-on experience in Designing / prototyping a Data science engagement on the chosen case study. You will be able to use the results in your day-to-day life.
We divide the data scientists into clickers and coders. Clickers are those data scientists who use a data science tool with a user interface to provide a high-level specification. Examples include SPSS Modeler, Excel, Alteryx and Ikigailabs. In each case you can add formula using pre-packaged libraries without writing code. The second set of data scientists are those who use a procedural language with libraries to write code for data science work. The objective of this course is to get you an introductory clicker experience in data science using Ikigailabs as a tool.
Related courses:
· If you are interested in a coding course, we offer a course using Python.
· Our data science methodology course is also designed for Business Analysts and Project Managers with limited development background.
· If you are interested in advanced machine learning, we have made recommendation for courses.
· If you are interested in model deployment, monitoring and control, we offer a course on AI governance and control.
Course starts with two critical activities
· Set up Environment - step by step instructions in preparing sandbox environment for your Ikigailabs exercises
· Data Science Methodology - to review key steps, tasks and activities associated with our data science methodology
After above section, this course introduces our 7-step data science methodology and use Python to explain each step using our real-life use case example. These 7 steps include
· Step 1: Describe Use Case to explain selected use case for data science work
· Step 2: Describe Data to describe Data Sources and explain data sets using Ikigailabs
· Step 3: Prepare Datasets to Prepare Data Sets using Ikigailabs
· Step 4: Develop Model will provide hands-on exercises in applying many AI modeling techniques on data sets such as clustering, and regression using Ikigailabs.
· Step 5: Evaluate Model will provide measurements to Evaluate your AI Model Results
· Step 6: Deploy Model will provide process for deploying your AI models.
· Step 7: Monitor model will provide process for continuous monitoring and evaluating your models in production
In this course, we will give you an opportunity to design a use case and then work on its implementation using Ikigailabs. You should download all data sets and sample code. Complete all assignment in each section of the course and submit your final notebook using instructions provided.
Who this course is for:
- This course is for anyone interested in becoming a data scientist such as students, business analysts, developers, testing professionals.
- There are several job categories where this course can be used as introductory material, such as data scientists, AI or automation engineer, test engineers, and knowledge engineers.
User Reviews
Rating
Neena Sathi
Instructor's Courses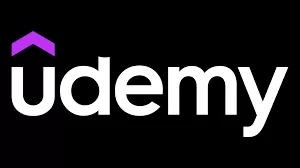
Udemy
View courses Udemy- language english
- Training sessions 42
- duration 4:46:57
- Release Date 2023/12/16