Data Mining - Unsupervised Learning
Elearning Moocs
10:42:12
Description
Data Mining - Unsupervised Learning
What You'll Learn?
- In Clustering or Segmentation, we reduce the number of rows. We have Hierarchical Clustering, Non-Hierarchical, Density-Based Clustering, Grid-based Clustering
- In Dimension Reduction, we reduce the number of columns. Linear Patterns are handled by Linear Discriminant Analysis, Non-negative Matrix Factorization.
- There is Collaborative Filtering in Recommendation System. Traditional Collaborative Filtering, Search-based Method, and Item-Item Collaborative Filtering.
- In Unsupervised Learning has 6 divisions which include Clustering, Dimension Reduction, Association Rules, Recommendation Syst
Who is this for?
What You Need to Know?
More details
DescriptionThe Data Mining - Unsupervised Learning course is designed to provide students with a comprehensive understanding of unsupervised learning techniques within the field of data mining. Unsupervised learning is a category of machine learning where algorithms are applied to unlabelled data to discover patterns, structures, and relationships without prior knowledge or guidance.
Throughout the course, students will explore various unsupervised learning algorithms and their applications in uncovering hidden insights from large datasets. The emphasis will be on understanding the principles, methodologies, and practical implementation of these algorithms rather than focusing on mathematical derivations.
The course will begin with an introduction to unsupervised learning, covering the basic concepts and goals. Students will learn how unsupervised learning differs from supervised learning and semi-supervised learning, and the advantages and limitations of unsupervised techniques. The importance of pre-processing and data preparation will also be discussed to ensure quality results.
The first major topic of the course will be clustering techniques. Students will dive into different clustering algorithms such as hierarchical clustering, k-means clustering, density-based clustering (e.g., DBSCAN), and expectation-maximization (EM) clustering. They will learn how to apply these algorithms to group similar data points together and identify underlying patterns and structures. The challenges and considerations in selecting appropriate clustering methods for different scenarios will be explored.
The course will then move on to dimensionality reduction, which aims to reduce the number of features or variables in a dataset while retaining relevant information. Students will explore techniques such as principal component analysis (PCA), singular value decomposition (SVD), and t-distributed stochastic neighbour embedding (t-SNE). They will understand how these methods can be used to visualize high-dimensional data and extract meaningful representations that facilitate analysis and interpretation.
Association rule mining will be another key topic covered in the course. Students will learn about the popular Apriori algorithm and FP-growth algorithm, which are used to discover interesting relationships and associations among items in transactional datasets. They will gain insights into evaluating and interpreting association rules, including support, confidence, and lift measures, and their practical applications in market basket analysis and recommendation systems.
The course will also address outlier detection, a critical task in unsupervised learning. Students will explore statistical approaches such as z-score and modified z-score, as well as distance-based approaches like the Local Outlier Factor and Isolation Forest. They will understand how to identify anomalies in data, which can provide valuable insights into potential fraud detection, network intrusion detection, or system failure prediction.
Evaluation and validation of unsupervised learning models will be an essential aspect of the course. Students will learn about internal and external evaluation measures, including silhouette coefficient, purity, and Rand index. They will gain skills in assessing the quality of clustering results and measuring the performance of dimensionality reduction techniques.
Throughout the course, students will be exposed to various real-world applications of unsupervised learning. They will discover how market segmentation can be achieved through clustering, enabling businesses to target specific customer segments effectively. They will also explore image and text clustering, which has applications in image recognition, document organization, and recommendation systems. The course will highlight anomaly detection, which plays a crucial role in identifying fraudulent transactions, network intrusions, or manufacturing defects. Lastly, students will learn how unsupervised learning powers recommender systems, providing personalized recommendations based on user behaviour and preferences.
Hands-on experience will be a significant component of the course. Students will work on practical exercises and projects, applying unsupervised learning algorithms to real-world datasets using popular data mining tools and programming libraries such as Python's scikit-learn or R's caret package. They will gain proficiency in pre-processing data, selecting appropriate algorithms, fine-tuning parameters, and interpreting and visualizing the results.
By the end of the course, students will have a solid understanding of unsupervised learning techniques, their practical applications, and the ability to leverage these methods to discover valuable insights and patterns from unlabelled data.
Who this course is for:
- IT professionals, including software developers and database administrators, can gain valuable skills by taking a Data Mining course.
- Researchers who want to explore and analyze large datasets, discover patterns, and generate insights can benefit from a Data Mining course to learn appropriate methodologies and techniques.
- Professionals who want to enhance their skills in data analysis, pattern recognition, and predictive modeling can enroll in a Data Mining course to learn new techniques and stay updated with industry trends.
- Students pursuing degrees in these areas can benefit from a Data Mining course to develop skills and knowledge related to analyzing and extracting insights from large datasets.
The Data Mining - Unsupervised Learning course is designed to provide students with a comprehensive understanding of unsupervised learning techniques within the field of data mining. Unsupervised learning is a category of machine learning where algorithms are applied to unlabelled data to discover patterns, structures, and relationships without prior knowledge or guidance.
Throughout the course, students will explore various unsupervised learning algorithms and their applications in uncovering hidden insights from large datasets. The emphasis will be on understanding the principles, methodologies, and practical implementation of these algorithms rather than focusing on mathematical derivations.
The course will begin with an introduction to unsupervised learning, covering the basic concepts and goals. Students will learn how unsupervised learning differs from supervised learning and semi-supervised learning, and the advantages and limitations of unsupervised techniques. The importance of pre-processing and data preparation will also be discussed to ensure quality results.
The first major topic of the course will be clustering techniques. Students will dive into different clustering algorithms such as hierarchical clustering, k-means clustering, density-based clustering (e.g., DBSCAN), and expectation-maximization (EM) clustering. They will learn how to apply these algorithms to group similar data points together and identify underlying patterns and structures. The challenges and considerations in selecting appropriate clustering methods for different scenarios will be explored.
The course will then move on to dimensionality reduction, which aims to reduce the number of features or variables in a dataset while retaining relevant information. Students will explore techniques such as principal component analysis (PCA), singular value decomposition (SVD), and t-distributed stochastic neighbour embedding (t-SNE). They will understand how these methods can be used to visualize high-dimensional data and extract meaningful representations that facilitate analysis and interpretation.
Association rule mining will be another key topic covered in the course. Students will learn about the popular Apriori algorithm and FP-growth algorithm, which are used to discover interesting relationships and associations among items in transactional datasets. They will gain insights into evaluating and interpreting association rules, including support, confidence, and lift measures, and their practical applications in market basket analysis and recommendation systems.
The course will also address outlier detection, a critical task in unsupervised learning. Students will explore statistical approaches such as z-score and modified z-score, as well as distance-based approaches like the Local Outlier Factor and Isolation Forest. They will understand how to identify anomalies in data, which can provide valuable insights into potential fraud detection, network intrusion detection, or system failure prediction.
Evaluation and validation of unsupervised learning models will be an essential aspect of the course. Students will learn about internal and external evaluation measures, including silhouette coefficient, purity, and Rand index. They will gain skills in assessing the quality of clustering results and measuring the performance of dimensionality reduction techniques.
Throughout the course, students will be exposed to various real-world applications of unsupervised learning. They will discover how market segmentation can be achieved through clustering, enabling businesses to target specific customer segments effectively. They will also explore image and text clustering, which has applications in image recognition, document organization, and recommendation systems. The course will highlight anomaly detection, which plays a crucial role in identifying fraudulent transactions, network intrusions, or manufacturing defects. Lastly, students will learn how unsupervised learning powers recommender systems, providing personalized recommendations based on user behaviour and preferences.
Hands-on experience will be a significant component of the course. Students will work on practical exercises and projects, applying unsupervised learning algorithms to real-world datasets using popular data mining tools and programming libraries such as Python's scikit-learn or R's caret package. They will gain proficiency in pre-processing data, selecting appropriate algorithms, fine-tuning parameters, and interpreting and visualizing the results.
By the end of the course, students will have a solid understanding of unsupervised learning techniques, their practical applications, and the ability to leverage these methods to discover valuable insights and patterns from unlabelled data.
Who this course is for:
- IT professionals, including software developers and database administrators, can gain valuable skills by taking a Data Mining course.
- Researchers who want to explore and analyze large datasets, discover patterns, and generate insights can benefit from a Data Mining course to learn appropriate methodologies and techniques.
- Professionals who want to enhance their skills in data analysis, pattern recognition, and predictive modeling can enroll in a Data Mining course to learn new techniques and stay updated with industry trends.
- Students pursuing degrees in these areas can benefit from a Data Mining course to develop skills and knowledge related to analyzing and extracting insights from large datasets.
User Reviews
Rating
Elearning Moocs
Instructor's Courses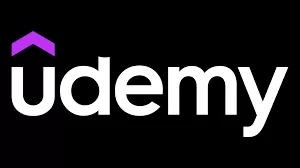
Udemy
View courses Udemy- language english
- Training sessions 57
- duration 10:42:12
- Release Date 2023/09/10