Building Clustering Models with scikit-learn
Janani Ravi
2:33:08
Description
This course covers several important techniques used to implement clustering in scikit-learn, including the K-means, mean-shift and DBScan clustering algorithms, as well as the role of hyperparameter tuning, and performing clustering on image data.
What You'll Learn?
Clustering is an extremely powerful and versatile unsupervised machine learning technique that is especially useful as a precursor to applying supervised learning techniques like classification. In this course, Building Clustering Models with scikit-learn, you will gain the ability to enumerate the different types of clustering algorithms and correctly implement them in scikit-learn. First, you will learn what clustering seeks to achieve, and how the ubiquitous k-means clustering algorithm works under the hood. Next, you will discover how to implement other techniques such as DBScan, mean-shift, and agglomerative clustering. You will then understand the importance of hyperparameter tuning in clustering, such as identifying the correct number of clusters into which your data ought to be partitioned. Finally, you will round out the course by implementing clustering algorithms on image data - an especially common use-case. When you are finished with this course, you will have the skills and knowledge to select the correct clustering algorithm based on the problem you are trying to solve, and also implement it correctly using scikit-learn.
More details
User Reviews
Rating
Janani Ravi
Instructor's Courses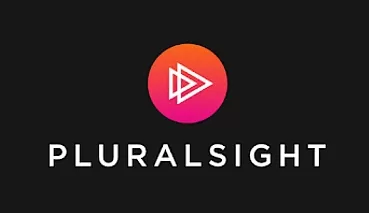
Pluralsight
View courses Pluralsight- language english
- Training sessions 38
- duration 2:33:08
- level average
- English subtitles has
- Release Date 2023/01/24