Big Data in Economics / Energy
Dr. Spyros Giannelos
2:21:58
Description
Big Data is everywhere in Finance, Energy, Machine Learning, A.I. etc. In this course we address them in various ways.
What You'll Learn?
- Big Data Methodology - Maximize Data Reduction & Minimize Loss of information. Application: Electricity demand
- Step-by-step: MATLAB, Excel, Python
- Creating a dummy Big Dataset
- Methodologies for comparing Big Data
- Summarizing Big Datasets
- Selecting fewer observations with set frequencies
- From Small Datasets to Big Data
- PowerQuery and Python for processing Big Data
- Rolling Average for Reducing Big Data
- Reducing Big Data using groupby, sum, min , etc
- The subtitles are manually created. Therefore, they are fully accurate. They are not auto-generated.
- Part of the giannelos dot com official certificate
Who is this for?
What You Need to Know?
More details
Description
What is the course about:
This course focuses on today's reality - the fact that all Datasets are getting Bigger and Bigger.
Big Data is becoming a big issue because they are reaching a point where these datasets cannot be processed.
In this course, we recognize this reality, by presenting a series of methodologies that enable us to deal with Big Data.
We begin with a 6-step methodology applied to a Big Dataset of Electricity Demand. We reduce it as much as possible,
while retaining the important information held in it.
We then proceed to create a Dummy Big-Dataset. This is necessary because many times companies prefer to conceal some information from inside their datasets.
Also, we proceed to select important information from within Big Data, and present a number of techniques to do so.
We also learn how to transition from small data , to big data, in an accurate way.
*** updating
Who:
I am a research fellow at Imperial College London, and I have been part of high-tech projects at the intersection of Academia & Industry for over 10 years, prior to, during & after my Ph.D. I am also the founder of the giannelos dot com program in data science.
Doctor of Philosophy (Ph.D.) in Analytics & Mathematical Optimization applied to Energy Investments, from Imperial College London, and Masters of Engineering (M. Eng.) in Power Systems and Economics.
Special Acknowledgements:
To Himalaya Bir Shrestha, energy system analyst, who has been contributing to the development of Python scripts for this course as well as to Medium.Â
Important:
Prerequisites: The course Data Science Code that appears all the time at Workplace.
Every detail is explained, so that you won't have to search online, or guess. In the end, you will feel confident in your knowledge and skills.
We start from scratch so that you do not need to have done any preparatory work in advance at all. Just follow what is shown on screen, because we go slowly and explain everything in detail.
Who this course is for:
- Enterpreneurs
- Economists
- Quants
- Members of the highly googled giannelos dot com program
- Investment Bankers
- Academics, PhD Students, MSc Students, Undergrads
- Postgraduate and PhD students.
- Data Scientists
- Energy professionals (investment planning, power system analysis)
- Software Engineers
- Finance professionals
What is the course about:
This course focuses on today's reality - the fact that all Datasets are getting Bigger and Bigger.
Big Data is becoming a big issue because they are reaching a point where these datasets cannot be processed.
In this course, we recognize this reality, by presenting a series of methodologies that enable us to deal with Big Data.
We begin with a 6-step methodology applied to a Big Dataset of Electricity Demand. We reduce it as much as possible,
while retaining the important information held in it.
We then proceed to create a Dummy Big-Dataset. This is necessary because many times companies prefer to conceal some information from inside their datasets.
Also, we proceed to select important information from within Big Data, and present a number of techniques to do so.
We also learn how to transition from small data , to big data, in an accurate way.
*** updating
Who:
I am a research fellow at Imperial College London, and I have been part of high-tech projects at the intersection of Academia & Industry for over 10 years, prior to, during & after my Ph.D. I am also the founder of the giannelos dot com program in data science.
Doctor of Philosophy (Ph.D.) in Analytics & Mathematical Optimization applied to Energy Investments, from Imperial College London, and Masters of Engineering (M. Eng.) in Power Systems and Economics.
Special Acknowledgements:
To Himalaya Bir Shrestha, energy system analyst, who has been contributing to the development of Python scripts for this course as well as to Medium.Â
Important:
Prerequisites: The course Data Science Code that appears all the time at Workplace.
Every detail is explained, so that you won't have to search online, or guess. In the end, you will feel confident in your knowledge and skills.
We start from scratch so that you do not need to have done any preparatory work in advance at all. Just follow what is shown on screen, because we go slowly and explain everything in detail.
Who this course is for:
- Enterpreneurs
- Economists
- Quants
- Members of the highly googled giannelos dot com program
- Investment Bankers
- Academics, PhD Students, MSc Students, Undergrads
- Postgraduate and PhD students.
- Data Scientists
- Energy professionals (investment planning, power system analysis)
- Software Engineers
- Finance professionals
User Reviews
Rating
Dr. Spyros Giannelos
Instructor's Courses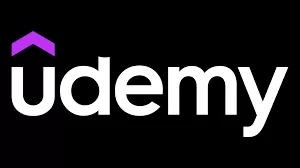
Udemy
View courses Udemy- language english
- Training sessions 14
- duration 2:21:58
- Release Date 2022/11/30