Become a TensorFlow Certified Professional Developer
Ligency Team,TFC Course
18:08:48
Description
Join the best training ground for AI mastery and gain the skills you need to become a TensorFlow Certified Developer.
What You'll Learn?
- Understand Deep Learning Fundamentals
- Construct three different deep learning models using TensorFlow and Keras
- Classify images using convolutional neural networks (CNNs) in TensorFlow.
- Apply image augmentation and transfer learning to enhance model performance.
- Utilize strategies to prevent overfitting, including augmentation and dropout.
- Process text through tokenization and sentence vector representation.
- Apply Recurrent Neural Networks (RNNs), Gated Recurrent Units (GRUs), and Long Short-Term Memory (LSTM) networks to NLP tasks
- Create Device-Based Models with TensorFlow Lite
Who is this for?
What You Need to Know?
More details
DescriptionIn this course you will learn everything you need to know to master the TensorFlow Developer Certification.
We will start by studying Deep Learning in depth so that you can understand how artificial neural networks work and learn. And while covering the Deep Learning theory we will also build together three different Deep Learning models in TensorFlow and Keras, from scratch, step by step, and coding every single line of code together.
Then, we will move on to Computer Vision, where you will learn how to classify images using convolutions with TensorFlow. You will also learn some techniques such as image augmentation and transfer learning to get even more performance in your computer vision tasks. And we will practice all this on real-world image data, while exploring strategies to prevent overfitting, including augmentation and dropout.
Then, you will learn how to use JavaScript, in order to train and run inference in a browser, handle data in a browser, and even build an object classification and recognition model using a webcam.
Then you will learn how to do Natural Language Processing using TensorFlow. Here we will build natural language processing systems, process text including tokenization and representing sentences as vectors, apply RNNs, GRUs, and LSTMs in TensorFlow, and train LSTMs on existing text to create original poetry and more.
And finally, you will also learn how to build Device-based Models with TensorFlow Lite. In this last part we will prepare models for battery-operated devices, execute models on Android and iOS platforms, and deploy models on embedded systems like Raspberry Pi and microcontrollers.
Who this course is for:
The course is targeted towards AI practitioners, aspiring data scientists, Tech enthusiasts, and consultants wanting to pass the TensorFlow Developer Certification. Hereâs a list of who is this course for:
Data Scientists who simply want to learn how to use TensorFlow at an advanced level.
Data Scientists who want to pass the TensorFlow Developer Certification.
AI Practitioners who want to build more powerful AI models using TensorFlow.
Tech enthusiasts who are passionate about AI and want to gain real-world practical experience with TensorFlow.
Course Prerequisites:
Basic knowledge of programming is recommended. Some experience in Machine Learning is also preferable. However, these topics will be extensively covered during early course lectures; therefore, the course has no prerequisites, and is open to anyone with basic programming knowledge. Students who enrol in this course will master data science fundamentals and directly apply these skills to solve real world challenging business problems.
*Terms & Conditions of Exam Guarantee:
Ligency Ventures Pty Ltd, U.K provides the following guarantee for the TensorFlow Developer Professional Certificate Course:
If you take your TensorFlow Developer Certificate exam within 30 days of enrolling and completing this course 100% and you sit the exam and receive a score above zero, but below the minimum score required to pass the exam, then Ligency Ventures Pty Ltd, U.K will pay for your second exam attempt provided the following conditions are met: you paid at least $1 for this course and it was not refunded, AND before sitting the exam, you diligently watched and followed along with all of the tutorials in the course (completed all case studies and have all codes under your Google Colab account), AND you completed all practical activities including but not limited to challenges within the sections, quizzes, homework exercises and all provided practice exams.
Ligency Ventures Pty Ltd may request evidence of fulfilling the above conditions, thereby it's important that you save your work when taking the course and doing the practical assignments.
Who this course is for:
- Data Scientists who simply want to learn how to use TensorFlow at an advanced level.
- Data Scientists who want to pass the TensorFlow Developer Certification.
- AI Practitioners who want to build more powerful AI models using TensorFlow.
- Tech enthusiasts who are passionate about AI and want to gain real-world practical experience with TensorFlow.
In this course you will learn everything you need to know to master the TensorFlow Developer Certification.
We will start by studying Deep Learning in depth so that you can understand how artificial neural networks work and learn. And while covering the Deep Learning theory we will also build together three different Deep Learning models in TensorFlow and Keras, from scratch, step by step, and coding every single line of code together.
Then, we will move on to Computer Vision, where you will learn how to classify images using convolutions with TensorFlow. You will also learn some techniques such as image augmentation and transfer learning to get even more performance in your computer vision tasks. And we will practice all this on real-world image data, while exploring strategies to prevent overfitting, including augmentation and dropout.
Then, you will learn how to use JavaScript, in order to train and run inference in a browser, handle data in a browser, and even build an object classification and recognition model using a webcam.
Then you will learn how to do Natural Language Processing using TensorFlow. Here we will build natural language processing systems, process text including tokenization and representing sentences as vectors, apply RNNs, GRUs, and LSTMs in TensorFlow, and train LSTMs on existing text to create original poetry and more.
And finally, you will also learn how to build Device-based Models with TensorFlow Lite. In this last part we will prepare models for battery-operated devices, execute models on Android and iOS platforms, and deploy models on embedded systems like Raspberry Pi and microcontrollers.
Who this course is for:
The course is targeted towards AI practitioners, aspiring data scientists, Tech enthusiasts, and consultants wanting to pass the TensorFlow Developer Certification. Hereâs a list of who is this course for:
Data Scientists who simply want to learn how to use TensorFlow at an advanced level.
Data Scientists who want to pass the TensorFlow Developer Certification.
AI Practitioners who want to build more powerful AI models using TensorFlow.
Tech enthusiasts who are passionate about AI and want to gain real-world practical experience with TensorFlow.
Course Prerequisites:
Basic knowledge of programming is recommended. Some experience in Machine Learning is also preferable. However, these topics will be extensively covered during early course lectures; therefore, the course has no prerequisites, and is open to anyone with basic programming knowledge. Students who enrol in this course will master data science fundamentals and directly apply these skills to solve real world challenging business problems.
*Terms & Conditions of Exam Guarantee:
Ligency Ventures Pty Ltd, U.K provides the following guarantee for the TensorFlow Developer Professional Certificate Course:
If you take your TensorFlow Developer Certificate exam within 30 days of enrolling and completing this course 100% and you sit the exam and receive a score above zero, but below the minimum score required to pass the exam, then Ligency Ventures Pty Ltd, U.K will pay for your second exam attempt provided the following conditions are met: you paid at least $1 for this course and it was not refunded, AND before sitting the exam, you diligently watched and followed along with all of the tutorials in the course (completed all case studies and have all codes under your Google Colab account), AND you completed all practical activities including but not limited to challenges within the sections, quizzes, homework exercises and all provided practice exams.
Ligency Ventures Pty Ltd may request evidence of fulfilling the above conditions, thereby it's important that you save your work when taking the course and doing the practical assignments.
Who this course is for:
- Data Scientists who simply want to learn how to use TensorFlow at an advanced level.
- Data Scientists who want to pass the TensorFlow Developer Certification.
- AI Practitioners who want to build more powerful AI models using TensorFlow.
- Tech enthusiasts who are passionate about AI and want to gain real-world practical experience with TensorFlow.
User Reviews
Rating
Ligency Team
Instructor's CoursesTFC Course
Instructor's Courses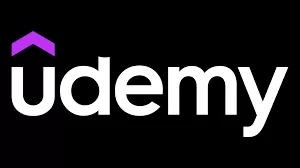
Udemy
View courses Udemy- language english
- Training sessions 152
- duration 18:08:48
- Release Date 2024/10/11