Bayesian Statistics: A Step-by-step Introduction
Brian Greco
5:32:53
Description
A former Google data scientist helps you master the basics of Bayesian statistics, with examples in R and Stan
What You'll Learn?
- Understand how Bayes' rule can be used to update beliefs
- Use conjugate priors and likelihoods to model binary, count, and continuous data
- Understand the concepts of prior distributions, posterior distributions, likelihood functions, and predictive distributions
- Understand how statistical software can be used to compute and visualize information about your beliefs
Who is this for?
What You Need to Know?
More details
DescriptionThis course teaches the foundational material of statistics covered in an introductory college course, with a focus on mastering the basic components of any Bayesian model - the prior distribution and the likelihood, and how to find a posterior distribution, credible intervals, and predictive distributions. Along the way, you'll become more comfortable with probability in general and gain a new perspective on how to analyze data!
We start from scratch - no experience in Bayesian statistics is required. Students should have a strong grasp of basic algebra and arithmetic. R and RStudio, or Python, is required if you would like to run the optional coding sections
The course includes:
5.5 hours of video lectures
Interactive demonstrations using R and Stan (Python code is included too!)
Quizzes to check your understanding
Review assignments with solutions to practice what you have learned
You will learn:
The basic rules of probability
Bayes' rule, including common examples with medical testing and flipping coins
The terminology of different components of a Bayesian model:Â the prior distribution, posterior, likelihood, and predictive distribution
Conjugate priors
Credible intervals and Bayes estimators
Modeling binary data with the Bernoulli and Binomial Distribution, and the Beta distribution prior
Modeling count data with the Poisson Distribution, and the Gamma distribution prior
Modeling continuous data with the Normal Distribution, and the Normal distribution prior
An introduction to simple linear regression
This course is ideal for many types of students:
Anyone who wants to learn the foundations of Bayesian statistics and understand concepts like priors, posteriors and credible intervals
Data science and data analytics professionals who would like to refresh and expand their statistics knowledge
Academics in the social, biological, and physical sciences
This course is ideal for anyone, from beginners to seasoned professionals. It doesn't matter if you're just starting your journey in data science, looking to upgrade your existing skills, or simply have an interest in Bayesian statistics. My goal is to make Bayesian statistics accessible and understandable for all.
Who this course is for:
- Current and aspiring data scientists and data analysts
- Academics in the social, biological, and physical sciences
- University students studying mathematics or statistics
- Anybody who wants to learn to rigorously update their beliefs from data.
This course teaches the foundational material of statistics covered in an introductory college course, with a focus on mastering the basic components of any Bayesian model - the prior distribution and the likelihood, and how to find a posterior distribution, credible intervals, and predictive distributions. Along the way, you'll become more comfortable with probability in general and gain a new perspective on how to analyze data!
We start from scratch - no experience in Bayesian statistics is required. Students should have a strong grasp of basic algebra and arithmetic. R and RStudio, or Python, is required if you would like to run the optional coding sections
The course includes:
5.5 hours of video lectures
Interactive demonstrations using R and Stan (Python code is included too!)
Quizzes to check your understanding
Review assignments with solutions to practice what you have learned
You will learn:
The basic rules of probability
Bayes' rule, including common examples with medical testing and flipping coins
The terminology of different components of a Bayesian model:Â the prior distribution, posterior, likelihood, and predictive distribution
Conjugate priors
Credible intervals and Bayes estimators
Modeling binary data with the Bernoulli and Binomial Distribution, and the Beta distribution prior
Modeling count data with the Poisson Distribution, and the Gamma distribution prior
Modeling continuous data with the Normal Distribution, and the Normal distribution prior
An introduction to simple linear regression
This course is ideal for many types of students:
Anyone who wants to learn the foundations of Bayesian statistics and understand concepts like priors, posteriors and credible intervals
Data science and data analytics professionals who would like to refresh and expand their statistics knowledge
Academics in the social, biological, and physical sciences
This course is ideal for anyone, from beginners to seasoned professionals. It doesn't matter if you're just starting your journey in data science, looking to upgrade your existing skills, or simply have an interest in Bayesian statistics. My goal is to make Bayesian statistics accessible and understandable for all.
Who this course is for:
- Current and aspiring data scientists and data analysts
- Academics in the social, biological, and physical sciences
- University students studying mathematics or statistics
- Anybody who wants to learn to rigorously update their beliefs from data.
User Reviews
Rating
Brian Greco
Instructor's Courses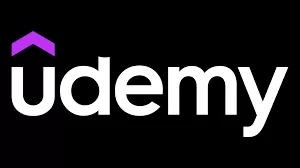
Udemy
View courses Udemy- language english
- Training sessions 48
- duration 5:32:53
- Release Date 2023/10/04