AI For Engineering Applications: A-Z
Dr.Mohammad Samara
12:03:47
Description
AI For Engineering Applications: A-Z
What You'll Learn?
- Understand the needed AI for Engineering Applications
- How to Code an Optimize model from scratch
- How to Code a K-Means Clustering from scratch
- How to Code a Q table Reinforcement Learning Engine from Scratch
- Use Google Or-Tools to optimize a plant scheduling problem.
- Use OpenAI baselines library to solve a control problem.
- Use Keras to construct a U-net neural network to segment (outline) a crack on a surface.
- Predict machine failure using real aircraft engine data.
Who is this for?
More details
DescriptionDescription
This is a complete course that will prepare you to use Machine Learning in Engineering Applications from A to Z. We will cover the fundamentals of Machine Learning and its applications in Engineering Companies, focusing on 4 types of machine learning: Optimization, Structured data, Reinforcement Learning, and Machine Vision.
What skills will you Learn:
In this course, you will learn the following skills:
Understand the math behind Machine Learning Algorithms.
Write and build Machine Learning Algorithms from scratch.
Preprocess data for Images, Reinforcement learning, structured data, and optimization.
Analyze data to extract valuable insights.
Use opensource libraries to apply Machine Learning to the different types of machine learning.
We will cover:
Fundamentals of Optimization and building optimization algorithms from scratch.
Use Google OR Tools optimization library/solver to solve Shop job problems.
Fundamentals of Structured Data processing algorithms and building data clustering using K-Nearest Neighbors algorithms from scratch.
Use scikit-learn library along with others to predict the Remaining Useful Life of Aircraft Engines (Predictive maintenance).
Fundamentals of Reinforcement Learning and building Q-Table algorithms from scratch.
Use Keras & Stable baselines libraries to control room temperature and construct a custom-made Environment using OpenAI Gym.
Fundamentals of Deep Learning and Networks used in deep learning for machine vision inspection.
The use of TensorFlow/ Keras to construct Deep Neural Networks and process images for Classification using CNN (images that have cracks and images that do not) and crack Detection and segmentation using U-Net (outline the crack location in every crack image).
If you do not have prior experience in Machine Learning or Computational Engineering, that's no problem. This course is complete and concise, covering the fundamentals of Machine Learning followed by using real data with strong opensource libraries needed to apply AI in Companies. Let's work together to fulfill the need of companies to apply Machine Learning in Engineering applications to MAKEÂ OURÂ FUTUREÂ ENGINEERINGÂ PRODUCTSÂ SMARTER.
Who this course is for:
- Engineers and Programmers whom want to get familiar with applying AI for Engineering applications
Description
This is a complete course that will prepare you to use Machine Learning in Engineering Applications from A to Z. We will cover the fundamentals of Machine Learning and its applications in Engineering Companies, focusing on 4 types of machine learning: Optimization, Structured data, Reinforcement Learning, and Machine Vision.
What skills will you Learn:
In this course, you will learn the following skills:
Understand the math behind Machine Learning Algorithms.
Write and build Machine Learning Algorithms from scratch.
Preprocess data for Images, Reinforcement learning, structured data, and optimization.
Analyze data to extract valuable insights.
Use opensource libraries to apply Machine Learning to the different types of machine learning.
We will cover:
Fundamentals of Optimization and building optimization algorithms from scratch.
Use Google OR Tools optimization library/solver to solve Shop job problems.
Fundamentals of Structured Data processing algorithms and building data clustering using K-Nearest Neighbors algorithms from scratch.
Use scikit-learn library along with others to predict the Remaining Useful Life of Aircraft Engines (Predictive maintenance).
Fundamentals of Reinforcement Learning and building Q-Table algorithms from scratch.
Use Keras & Stable baselines libraries to control room temperature and construct a custom-made Environment using OpenAI Gym.
Fundamentals of Deep Learning and Networks used in deep learning for machine vision inspection.
The use of TensorFlow/ Keras to construct Deep Neural Networks and process images for Classification using CNN (images that have cracks and images that do not) and crack Detection and segmentation using U-Net (outline the crack location in every crack image).
If you do not have prior experience in Machine Learning or Computational Engineering, that's no problem. This course is complete and concise, covering the fundamentals of Machine Learning followed by using real data with strong opensource libraries needed to apply AI in Companies. Let's work together to fulfill the need of companies to apply Machine Learning in Engineering applications to MAKEÂ OURÂ FUTUREÂ ENGINEERINGÂ PRODUCTSÂ SMARTER.
Who this course is for:
- Engineers and Programmers whom want to get familiar with applying AI for Engineering applications
User Reviews
Rating
Dr.Mohammad Samara
Instructor's Courses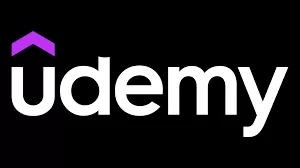
Udemy
View courses Udemy- language english
- Training sessions 52
- duration 12:03:47
- Release Date 2023/05/05