Advanced Text Analytics: Topic Modeling and Named Entity Recognition
Ria Cheruvu
40:09
Description
Learn how to use advanced topic modeling and named entity recognition (NER) for text analytics, covering the math and code behind topic modeling and NER algorithms (e.g., transformer-based) and how to integrate both in your workflows.
What You'll Learn?
As our primary mode of communication, text surrounds us -- in books, articles, social media posts, reviews, emails, and more. By leveraging text analytics, we can extract meaningful insights from this data and make intelligent decisions (for example, via sentiment analysis).
In this course, Advanced Text Analytics: Topic Modeling and Named Entity Recognition, you’ll gain the theory and practical implementation skills to apply advanced topic modeling and named entity recognition (NER) techniques for real-world use cases.
First, you’ll explore the motivation and concept behind using topic modeling for discovering patterns and themes in text. Next, you'll dive deeper into the mathematical and programmatic implementation of a popular topic-modeling algorithm, latent Dirichlet allocation (LDA). Then, you’ll learn how to implement NER, including its algorithms such as conditional random fields, transformer-based methods, and more. Finally, you'll look at how to combine topic modeling and NER for analyzing complex texts, and how to draw insights from the results.
When you’re finished with this course, you’ll have the skills and knowledge of evaluating and applying advanced topic modeling and named entity recognition algorithms needed to perform text analytics for your use cases.
More details
User Reviews
Rating
Ria Cheruvu
Instructor's Courses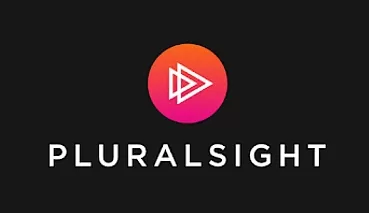
Pluralsight
View courses Pluralsight- language english
- Training sessions 12
- duration 40:09
- level average
- English subtitles has
- Release Date 2024/04/29